Computing Commons
Designing public compute for people and society
7 February 2025
Reading time: 89 minutes
Executive summary
Compute, or computational power, has emerged as a fundamental currency of AI technologies, shaping not only the technical capabilities of AI systems and who is able to build them, but also the competitive dynamics and strategic landscape of the AI sector. As AI models grow increasingly sophisticated, access to and control of large-scale compute resources have become critical determinants of research progress, commercial success and ultimately who can participate in AI development.
This report focuses on ‘public compute’, which we define loosely as initiatives which use government funds to provide particular groups with access to compute resources. This can take a variety of forms, from the provision of hardware (for example, graphics processing units or GPUs) or vouchers for accessing private cloud services, to direct access to public supercomputing projects operated by government or state-funded entities.
The announcement of the multibillion-dollar Stargate Project for data centre investment in the US, the publication of the AI Opportunities Action Plan in the UK, and live debate over the effect of the DeepSeek R1 model on future compute demand all speak to the urgency of better understanding the impact of compute availability. With increasing calls for ‘public’ alternatives to corporate infrastructure at all levels of the ‘AI stack’, compute – as a central input for modern AI development – is necessarily part of this picture. At the centre of this debate is how we can evaluate the extent to which different approaches result in broader public benefit, a key concern of this month’s AI Action Summit in Paris.
This report summarises the findings of a research project carried out by the Ada Lovelace Institute (Ada), with the support of the Mozilla Foundation. Based on interviews with policymakers and experts across multiple jurisdictions, we map existing public compute initiatives and provide recommendations for policymakers looking to scope and implement such policies.
Key findings
As AI has emerged as an industrial priority globally, state provision of computing resources has gained renewed prominence. While some countries are developing new AI-focused initiatives like the National AI Research Resource in the US and the AI Research Resource in the UK, others are adapting existing high-performance computing (HPC) programmes to meet evolving needs. Our analysis reveals significant diversity in how these initiatives have been conceived and delivered.
Most existing initiatives primarily serve research communities rather than broader public interest applications. While this reflects the historical development of public computing infrastructure, this focus may limit the potential for these investments to shape the development of AI in the public interest. There are also concerns that without careful policy design, public compute investments risk entrenching existing market concentration in AI development and contributing to the environmental harms associated with the growth in data centre demand globally.
Four families of public compute provision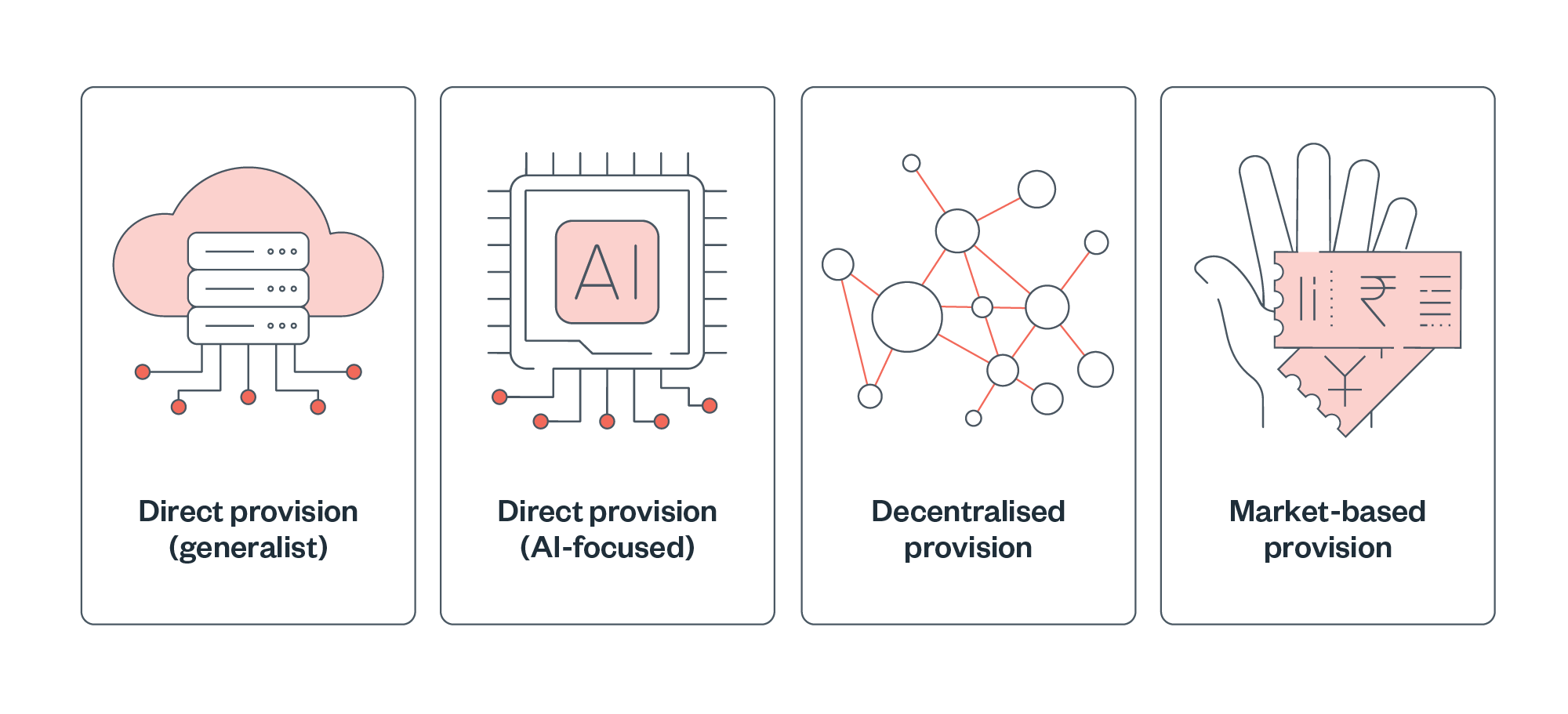
We identify four broad families of public compute provision: direct provision for AI and for general purposes, decentralised provision and market-based provision.
Direct provision (generalist) | Direct provision (AI-focused) | Decentralised provision | Market-based provision | |
Description | Large-scale, general-purpose infrastructure aimed at servicing multiple use cases – from academic research to public-sector projects – through flexible architecture.
Typically involves significant capital investment in facilities housed within national laboratories or research institutions.
|
Infrastructure specifically designed for computationally intensive AI workloads such as ‘frontier’ LLM development, multi-modal and multi-agent systems.
These initiatives are typically GPU-centric and optimised for AI workloads, with more flexible access patterns and integrated AI-specific support services. |
The use of public resources to facilitate networks of smaller facilities distributed across regions. These facilities can themselves be publicly- or privately-owned.
|
The use of public funds to provide financial incentives, vouchers or subsidies to help users access compute from existing commercial providers.
|
Examples | US Department of Energy supercomputers; European High-Performance Computing (EuroHPC) Joint Undertaking | AI Research Resource (UK); National AI Research Resource (US) | Open Cloud Compute (India); China’s network of municipally-owned datacentres | Compute vouchers provided as part of India’s IndiaAI mission |
Effective for | 1. Supporting scientific research
2. Supporting small and medium enterprises 3. Digital sovereignty for critical uses like military or strategic communications |
1. Supporting AI research, including AI safety research
2. Supporting the domestic AI industry 3. AI sovereignty 4. Market shaping |
1. Market shaping
2. Supporting the domestic compute industry |
1. Supporting the domestic AI industry
2. Supporting small and medium AI enterprises 3. Supporting scientific research |
Potential drawbacks | Risk of spreading investment too thinly across different types of compute.
Usually takes the form of large projects, which present coordination and hardware specification difficulties. |
Risk of entrenching monopolies when not paired with market shaping measures. | Large scale versions have yet to be tried: it is not clear that decentralisation can work at national scales with very large investments.
Ignores chip design and manufacturing layers. |
Risk of simply subsidising incumbent companies.
Does not tackle dependencies in the compute supply chain and cannot on its own contribute to digital or AI sovereignty. |
Recommendations
Across these families of compute provision, we find common challenges around value capture, strategic coherence, flexibility and environmental impact. We make detailed recommendations across each of these areas towards the end of the report:
To avoid value capture:
- Insulate public compute strategies from industry lobbying.
- Target procurement as part of a holistic industrial strategy for compute.
- Explore conditionality for user access to compute.
- Provide adjacent resources to ensure a wide range of organisations can benefit.
- Consider complementary interventions to address market concentration.
To achieve strategic coherence:
- Ensure mechanisms to coordinate on a national level while preserving local autonomy.
- Develop integrated strategies for compute infrastructure and skills.
- Coordinate for energy and water requirements.
To balance flexibility and longevity:
- Set long-term targets and funding envelopes, but with flexibility on delivery.
- Build modular software infrastructure alongside hardware.
To square compute investments with environmental goals:
- Review the impact of AI on data centre demand, including the role of public compute initiatives.
- Require environmental commitments from suppliers and users.
Glossary
Cloud: Computing resources (like processing power, storage and software) accessed remotely over the internet rather than on local computers. Cloud services are typically provided by large technology firms like Amazon, Google and Microsoft.
Compute: The resources required to perform computational tasks, including hardware (like processors and memory), software and supporting infrastructure. In the context of AI, compute typically refers to the processing power needed to train and run AI models.
CPU (central processing unit): The primary processor in a computer that performs most basic computations. While effective for general computing tasks, CPUs are less efficient than GPUs for the parallel processing needed in AI applications.
Exascale: A measure of computing speed – specifically, the ability to perform at least one quintillion (10^18) calculations per second. Exascale computers represent the current frontier of supercomputing capability.
FLOPS (floating point operations per second): A measure of computer performance, specifically how many numerical calculations a system can perform each second. Modern supercomputers are often measured in petaFLOPS (10^15 FLOPS) or exaFLOPS (10^18 FLOPS).
GPU (graphics processing unit): Specialised processors originally designed for rendering graphics but now widely used in AI applications due to their ability to perform many calculations simultaneously. GPUs are particularly important for training large AI models.
HPC (high-performance computing): The use of supercomputers and parallel processing techniques to solve complex computational problems. HPC systems are typically used for scientific research, climate modelling and other computationally intensive tasks.
Large language models (LLMs): LLMs are trained on significant amounts of text data, enabling them to generate natural language responses to a wide range of inputs. LLMs are used to perform a wide range of text-based tasks, such as answering questions, autocompleting text, translating and summarising, in response to a wide range of inputs and prompts.
Modelling: The use of computers to simulate and analyse complex systems or phenomena, such as weather patterns, molecular interactions or economic scenarios. Scientific modelling often requires significant computing power.
Public compute: Initiatives that use government funds to provide access to computing resources for particular groups or purposes. This can include direct provision of hardware, voucher schemes or partnerships with private providers.
Public funds: Money collected and spent by government bodies, typically through taxation, to provide public services and infrastructure.
Public goods: Products or services that benefit society as a whole and are typically difficult to provide through market mechanisms alone. Classic examples include street lighting or clean air.
Public orientation: An approach to technology development that prioritises broad public benefit over private profit, often emphasising accessibility, accountability and alignment with societal needs.
Public ownership: Direct government ownership and control of assets or infrastructure, as opposed to private ownership or public-private partnerships.
Public-private partnerships: Collaborations between government entities and private companies to deliver public services or infrastructure. In the context of compute, these often involve government funding combined with private sector expertise and resources.
Voucher schemes: Programmes where governments provide credits or certificates that recipients can use to purchase services from approved providers. In the context of compute, these often allow researchers or companies to access commercial cloud computing services.
How to read this report
This report is designed to offer a foundation for those engaged in thinking about the public provision of compute resources, and to provide a framework with which to understand the different emerging public compute for AI initiatives globally. We therefore suggest reading the report in its entirety to gain a comprehensive understanding of public compute and the role it plays within the global AI ecosystem. However, we have suggested sections to prioritise.
If you are new to the term public compute or interested in learning what it is:
- Read the ‘Introduction’, the ‘Public compute initiatives in context’ section and ‘Appendix – Case studies’ for an understanding of what we mean by ‘public compute’, a brief history of these initiatives and to know more about current activity in leading countries globally.
If you are a policymaker working on the design of public compute issues:
- For an overview of the report, read the ‘Executive summary’ and ‘Introduction’.
- Read the section ‘Public compute initiatives today’ and ‘Appendix – Case studies’ for an overview of the variety of approaches currently taken to public compute delivery.
- Read the section ‘Design options for public compute’ to understand how these different approaches can be categorised into four broad ‘families’.
- Read the ‘Recommendations for the future development of public compute policies’ section for a discussion of the key challenges that exist in this area of policy, and our suggestions for how they can be navigated.
If you are a researcher, civil society organisation or member of the public interested in public compute:
- Read the ‘Introduction’, the ‘Public compute initiatives in context’ section and ‘Appendix – Case studies’ for an understanding of what we mean by ‘public compute’, a brief history of these initiatives and to know more about current activity in leading countries globally.
- Read the ‘Recommendations for the future development of public compute policies’ section for a discussion of the key challenges that exist in this area of policy, and our suggestions for how they can be navigated.
Methodology and evidence base
Where not otherwise cited, the claims made in this report are derived from three sources:
- Desk research carried out by the authors.
- Interviews with more than 20 policymakers and experts working on the design, delivery and scrutiny of public compute in jurisdictions including Canada, the EU, India, the UK and the USA.
- Two expert roundtables with civil society and academic experts.
For a full list of interviewees and roundtable attendees, see the ‘Acknowledgements’ section at the end of the report.
Introduction
Compute, or computational power, has emerged as a fundamental currency of AI technologies, shaping not only the technical capabilities of AI systems and who is able to build them, but also the competitive dynamics and strategic landscape of the AI sector. As AI models grow increasingly sophisticated, access to and control of large-scale compute resources have become critical determinants of research progress, commercial success and ultimately who can participate in AI development.
State provision of computing resource is a well-established area of policy. As early as the late twentieth century, governments invested in national computing resources for academic, national security and public interest use cases including weather forecasting and climate research. Many of the first ‘supercomputers’ were procured by public organisations, and today supercomputers operated by the US government and the EU are among the fastest in the world.[1]
The emergence of AI as an industrial priority for countries and trading blocs across the world has raised the prominence of the issue of compute access. New AI-focused initiatives such as the UK’s AI Research Resource and the US’s National AI Research Resource pilot aim to provide world-class compute to domestic researchers. The UK has also set out significant ambitions to grow public and private compute capabilities in its AI Opportunities Action Plan. Long-established programmes such as the EU’s European High-Performance Computing (EuroHPC) Joint Undertaking are establishing new AI-related priorities. This activity extends beyond the UK, USA and EU, with countries like China and India also making significant investments in computing resources.
In this paper, we use the term ‘public compute’ to refer to this broad family of policies, defined loosely as initiatives which use government funds to provide particular groups with access to compute resources. This can take a variety of forms, from the provision of hardware (for example, graphics processing units or GPUs) or vouchers for accessing private cloud services, to direct access to public supercomputing projects operated by government or state-funded entities.
Initiatives may also aspire to be public in other ways, beyond their means of funding. For example, they may provide open, public access to compute or promote particular ‘public interest’ research areas. For the purposes of this report, our aim was to understand ‘public compute’ as initiatives which use government funds, and so these contrasting definitions were not considered strict criteria for inclusion in our analysis.
Proponents of public compute maintain that these investments are necessary for countries to remain competitive in the context of ‘arms race’ dynamics between states looking to develop advanced AI. Some campaigners and industry voices suggest that these could help to broaden access to the resources needed to train and deploy AI systems, bridging the compute divide between the largest tech companies and smaller companies or research institutions. With increasing calls for public alternatives to corporate infrastructure at all levels of the ‘AI stack’, compute – as a central input for modern AI development – is necessarily part of this picture.[2]
That said, there is a sense in which these aims – national competitiveness on the one hand and a greater diversity of public interest applications on the other – can be understood as in tension. Critics have raised concerns about the scale of public compute investment given the lack of genuinely independent alternatives to AI infrastructures operated by the largest technology firms, which are overwhelmingly headquartered in the USA and China.[3]
Against this backdrop, well-intentioned efforts to develop sovereign AI capabilities risk merely entrenching the market power of dominant players, and the geopolitical influence of the two preeminent AI superpowers. The Ada Lovelace Institute (Ada) explored some of these issues in a blog post[4] with Common Wealth[5] last year, highlighting the need for public compute investments to be made within a broader framework of market shaping policies if they are to mitigate these risks and yield genuine public benefit. There is also a sense in which national competitiveness – hedging against risks and deriving appropriate benefits from AI including through innovation – could be better served by more public interest applications.
This report is the culmination of a research project carried out by Ada, with the support of the Mozilla Foundation. Our research maps the existing and planned strategies for the public provision of compute with the aim of better understanding the design options available to national policymakers scoping and implementing public compute policies. It sets out four broad families of design options for public compute provision and makes recommendations for the future development of these policies.
Public compute initiatives in context
Public compute is a contested term with a complicated history. This section briefly defines our use of the term, before giving an overview of public compute initiatives up to the present day.
Defining public compute
Compute, or computational power, is used for many applications, but has become most prominent in recent years as a crucial part of the AI supply chain. It is often referred to as a discrete layer within this supply chain, but this layer can include a number of different components and operations.
Aligning with the description in Computational Power and AI, we define compute systems as comprising a stack of hardware, software and infrastructure components:[6]
- Hardware: chips such as graphics processing units (GPUs), originally used to render graphics in video games but now increasingly applied in AI due to their ability to support complex and parallel mathematical computations. Chips like central processing units (CPUs) are also used for both AI and non-AI applications, depending on the type of model being trained or run.
- Software: to manage data and enable the use of chips, and specialised programming languages for optimising chip usage. One example of compute software is Nvidia’s CUDA, which is required for using Nvidia’s GPUs.
- Infrastructure: other physical components of data centres such as cabling, servers and cooling equipment. Servers bring several chips together, and data centres are collections of servers.
As the rapid growth in the use of AI models has renewed interest in public compute, we approach AI-relevant compute as a way to understand larger questions of public compute provision. This means that much of the focus of this report is on AI-relevant compute. However, other kinds of public compute are also explored, such as ‘generalist’ models of provision that aim to support not only AI projects but broader categories of scientific research and technological development.
In this paper, we use the term ‘public compute’ to refer to this broad family of policies, defined loosely as initiatives which use government funds to provide particular groups with access to compute resources. This can take a variety of forms, from the provision of hardware (for example, graphics processing units or GPUs) or vouchers for accessing private cloud services, to direct access to public supercomputing projects operated by government or by state-funded entities.
Contested meanings of ‘public’
In this report, we primarily use ‘public’ to refer to the use of public funds to provide access to compute. Beyond their means of funding, public compute initiatives may also be ‘public’ in other ways. For example, the Mozilla Foundation has articulated three criteria for ‘public AI’:[7]
- Public goods: ‘Public AI should create open, accessible public goods and shared resources at all levels of the AI stack, especially where cost, scarcity and private lock-up pose the greatest barrier to public participation.’
- Public orientation: ‘Public AI should centre the needs of people and communities, especially those most underserved by market-led development, such as workers and marginalised groups.’
- Public use: ‘Public AI should prioritise AI applications in the public interest, especially those neglected by commercial incentives and those posing ethics or security concerns that make them irresponsible to pursue via private development.’
The Public AI network offers three further criteria in its white paper.[8] They are not the same as Mozilla’s, but there are some overlaps:[9]
- Public access: ‘Certain capabilities are so important for participation in public life that access to them should be universal. Public AI provides affordable access to these tools so that everyone can realise their potential.’
- Public accountability: ‘Public AI earns trust by ensuring ultimate control of development rests with the public, giving everyone a chance to participate in shaping the future.’
- Permanent public goods: ‘Public AI is funded and operated in a way to maintain the public goods it produces permanently, enabling innovators to safely build on a firm foundation.’
One final criterion that has been advocated for in relation to compute, and for AI more generally, is public ownership. Common Wealth, for example, has argued that AI itself, and not just its products, should be owned by the public so that they may decide the trajectory of its development and use.[10]
We offer these contrasting definitions as relevant points of comparison and many of the features they denote are present in the initiatives we analysed as part of this research. For example, we discuss questions of public orientation and public ownership below. However, for the purposes of this report, our aim was to understand ‘public compute’ as initiatives which use government funds, and especially in terms of how policymakers, designers and operators understand it. Therefore these contrasting definitions were not considered strict criteria for inclusion in our analysis.
Early public compute initiatives
The early history of supercomputing was deeply intertwined with government investment and priorities, particularly in the US. The first supercomputers were developed largely through defence funding, with early machines like the CDC 6600 and ILLIAC IV supported by agencies like the Defense Advanced Research Projects Agency (DARPA) and used extensively at national laboratories.[11]
The roots of public-sector computing lie primarily in scientific research and national security applications. From the late twentieth century onwards, governments – particularly in advanced economies – began establishing national supercomputing facilities, predominantly to serve the research community and strategic state functions.
In the US, this manifested through the Department of Energy’s (DOE) national laboratory system. Facilities like Oak Ridge and Argonne became home to some of the world’s most powerful supercomputers, supporting everything from climate modelling to nuclear weapons simulation.[12] This infrastructure was designed for highly specialised scientific workloads, particularly physics simulations and other computationally intensive modelling tasks.
The European approach developed differently, with more emphasis on distributed networks of computing resources. Initiatives such as the Partnership for Advanced Computing in Europe (PRACE), established in 2010, and later the European High-Performance Computing (EuroHPC) Joint Undertaking, established in 2018, exemplified this model. They created a pan-European supercomputing infrastructure that researchers could access across national boundaries.[13] However, individual European nations also continued to maintain their own national facilities.
In the UK, public computing provision centred around successive generations of the ARCHER supercomputer service, managed by the Edinburgh Parallel Computing Centre (EPCC) at the University of Edinburgh.[14]
This traditional model of public computing had several distinctive characteristics:
- It was highly centralised, with a small number of very large facilities.
- Access was heavily restricted, primarily serving academic researchers and government agencies.
- The focus was on specialised scientific workloads rather than general-purpose computing.
- Procurement cycles were long (often over five years) and focused on CPU-based architectures rather than the GPU-based architectures used today for AI training and inference.
The limitations of this model became increasingly apparent with the rise of data science and early machine learning applications in the 2010s. These new workloads required different architectures and more flexible access models, which in turn pushed established institutions to evolve.
However, the real paradigm shift would come with the emergence of foundation models and other compute-intensive forms of AI in the late 2010s and early 2020s.[15] This created new demands that the traditional public computing infrastructure was ill-equipped to meet – driving the current wave of AI-focused computing initiatives we see today.
This history helps explain some of the tensions in current public computing policy. Many of today’s initiatives are trying to bridge the gap between traditional high-performance computing (HPC) infrastructure – with its emphasis on scientific computing – and the more diverse computing needs of the AI era. Understanding this context is crucial for policymakers as they design new frameworks for public computing provision.
Large language models and the compute divide
The modern era of foundation models began with Google’s introduction of the Transformer architecture in 2017.[16] This breakthrough enabled models to process natural language data far more effectively than previous approaches. However, the inflection point came with OpenAI’s GPT series, which demonstrated that dramatic improvements in performance could be achieved primarily by scaling up model size and training compute.
This led to what is sometimes called the ‘scaling era’ in AI, characterised by rapid growth in model size, the number of parameters (the numerical values that a model learns during training that determine how input data is processed) and compute requirements. A survey of the size of best-in-class foundation models in recent years illustrates this point:[17]
- GPT-2 (2019): ~1.5 billion parameters
- GPT-3 (2020): 175 billion parameters
- PaLM (2022): 540 billion parameters
- GPT-4 (2023): Parameter count undisclosed but estimated to be substantially larger
Each step up in scale required exponentially more computing power. For context, training GPT-3 is estimated to have required thousands of GPU years of compute time, costing millions of dollars. This level of resource requirement created what researchers have called a ‘compute divide’ between a handful of well-resourced tech companies and other actors.[18]
The implications became particularly acute from 2022 onwards, as the success of ChatGPT led to a boom in the valuation of AI companies – even as those companies continued to post significant quarterly losses. This created a scramble for computing resources that has particularly affected researchers and smaller organisations, who have struggled to secure even modest resources. Instead they have formed dependencies on the infrastructure of major cloud computing and hardware companies like Nvidia, Google, Amazon and Microsoft.[19]
In jurisdictions such as China, scarcity has been exacerbated by supply chain constraints and export controls affecting the supply of high-end AI chips.[20] Within China, the impacts of export controls tend to affect start-ups rather than the larger firms who are able to stockpile the very best chips. The high global prices of advanced GPUs mean that this dynamic is perceptible even in countries not targeted by US-led export controls – further entrenching the compute divide both within and between nations.
Recent developments point towards a possible move away from scaling, with companies and entire national AI sectors incentivised to reduce compute intensity as a consequence of the compute divide. DeepSeek, a Chinese enterprise, has released two models in quick succession that achieve frontier model performance with a fraction of the compute that has so far been required to train frontier models.[21] In China’s case, constraints on compute availability have thus served as an impetus for innovation. Since elements of DeepSeek’s models are open sourced (including for commercial use), this innovation can potentially mean that compute requirements around the world could be transformed.
At the time of writing, it is still too early to determine the impact of low-compute frontier models on the AI landscape. Some commentators maintain that compute access will continue to serve as a competitive edge for AI developers.[22] That the biggest tech firms and their investors continue to believe this is perhaps best evidenced by the hundreds of billions of investment pledged to compute infrastructure in 2025 so far in the US alone.[23]
The status quo is a market where compute access has become a key determinant of who can participate in AI development.
For researchers and universities, this means being effectively locked out of computationally-intensive AI research such as much frontier LLM development is today.[24] As well as having direct effects on the viability of research projects in the public sector, it also has second-order effects in the form of driving AI and computer science talent from academia to private industry in the pursuit of ambitious projects, weakening independent research capacity and third-party assessments.[25] For start-ups, non-profits and smaller companies, this divide poses a significant obstacle to market entry: even when they have innovative ideas or approaches, they often cannot access the resources needed to develop them.[26]
For policymakers, this concentration raises several concerns:
- National competitiveness: Countries worry about falling behind their peers in compute access and related scientific fields. The UK, for instance, has fallen to 14th in the world supercomputer ranking, which ranks the most powerful computer systems in the world.[27]
- Strategic autonomy or digital/AI sovereignty: There is growing agreement among policymakers that compute access is crucial for sovereign AI capabilities, particularly for sensitive public-sector applications.[28] This is particularly acute in Europe, where concerns about dependence on US tech companies are prominent.[29] Concerns about dependence on US suppliers are also growing in the Global South.[30]
- Public interest research, applications and projects: Without public compute resources, important research in areas like AI safety or public sector applications might be neglected because they do not align with commercial priorities. Under the current market-based paradigm for AI development, access to and gains from AI research and development are typically privatised despite compelling reasons for open distribution and use.
The rise of the compute divide helps explain why public compute has become such a pressing policy issue – it is not just about supporting research, but about ensuring broader participation in an increasingly important technological field.
Public-sector responses to the compute divide
The rise of the compute divide in AI has driven various policy responses: the National AI Research Resource (NAIRR) in the US and the AI Research Resource (AIRR) in the UK are prominent examples of policymakers looking to provide researcher access to compute resources. However, there is ongoing debate about the right approach, and initiatives vary widely in their aims and delivery models. Some, for example, involve the direct provision of state-owned and/or operated compute resource to eligible organisations, while others use credit and voucher schemes to expand access to compute from existing private providers.
The policy challenge is further complicated by rapid technological change. Hardware capabilities and requirements evolve quickly, making it difficult for traditional government procurement cycles to keep pace.
Despite these challenges, there is growing consensus among policymakers that some form of public compute provision is necessary: the UK Government’s recent AI Opportunities Action Plan, for instance, and the bipartisan support in the US for the National AI Research Resource pilot, illustrate this well.
The question is increasingly not whether to provide public compute resources, but how to do so effectively while ensuring public benefit.
This has led to experimentation with different models – from direct provision of hardware to voucher schemes and public-private partnerships.
The remainder of this report surveys these public compute initiatives, before setting out four broad families of design options for public compute provision. We also make recommendations for the future development of these policies.
Public compute initiatives today
This section provides an overview of the various features of current public initiatives. For a list of case studies, see Appendix – Case studies’.
Aims
Existing public compute initiatives pursue various objectives, often simultaneously. These commonly include the following:
- Supporting scientific research and innovation in general, or in specific research domains (for example, climate science and drug discovery).
- Ensuring that advances in AI research are not automatically privatised because only large private corporations can access the required compute to train or deploy AI, and that benefits can accrue to the public.
- Increasing access to AI research capabilities beyond the private sector.
- Building strategic technological capabilities (for example, in the semiconductor supply chain) through procurement.
- Supporting domestic industry, small enterprises and start-ups carrying out fundamental AI research.
As this list indicates, most of the public compute initiatives that featured in our analysis are focused on research and development. Other aims that have been advocated for by proponents of public compute – such as public interest applications of AI and the creation of ‘open, accessible public goods and shared resources’ – remain underserved by these existing initiatives.
Traditional research computing infrastructure, exemplified by facilities like ARCHER2 in the UK, primarily focuses on supporting academic research and large-scale scientific modelling. However, interviewees for this report emphasised that newer initiatives often have broader ambitions around AI development and innovation. The National AI Research Resource (NAIRR) pilot in the US, for instance, explicitly aims to ‘democratise’ access to AI by providing users with the compute, skills and other resources they need to carry out AI research.
There is also a focus on AI development and applications that are not related to LLMs. India’s initiatives emphasise building technological capabilities in strategic sectors and supporting the development of domestic inputs to global compute supply chains. In China, multiple public-sector initiatives aim to provide compute specifically for AI model training purposes. In Singapore, public compute initiatives hope to uncover AI use cases beneficial for the public.
User groups
Different public compute approaches use different eligibility criteria to determine who can use resources provided. Among the programmes we studied, these have included:
- public, private or non-profit status
- organisation size
- national or regional status
- academic or scientific merit of user institution
- potential for research to contribute to innovation in strategic areas.
In practice, our interviews revealed that academic researchers remain the primary users of many public compute resources, though there is a trend towards supporting wider access. Most public compute initiatives studied provide access to entities within the national or regional jurisdiction of operation. The EU’s European High-Performance Computing (EuroHPC) Joint Undertaking initiative, for example, reserves 50 per cent of resources for EU-based researchers while giving member states flexibility to control allocation of the remaining capacity. India’s initiatives target both research institutions and private-sector entities, though interviewees noted the challenges in defining appropriate access criteria and ensuring equitable distribution of resources. Saudi Arabia requires start-ups to be registered within the country to qualify for access to subsidised compute access.
There is sometimes a gap between the targeted user base imagined by a public compute policy and the actual user base the government is able to reach. The risk here is that of continuing to benefit a small set of researchers or businesses that are already familiar with using public compute resources, rather than spreading the benefits over a wider base and supporting more diverse research.
Hardware procurement
Jurisdictions vary significantly in how much of the ‘compute stack’ they provide. Initiatives we studied include the following:
- Hardware-only support: direct provision of chips or computing resources.
- Hardware and software combinations: providing compute resources with necessary software tools.
- Enabling infrastructure provision: focusing on land, power and other supporting elements.
- Full-stack provision: comprehensive computing services including all elements above.
Media coverage of public compute initiatives often focuses on massive-scale compute installations. Traditional high-performance computing (HPC) users in academia often need large-scale systems optimised for modelling and simulation work, which have different architectural requirements than workloads for training or running AI models.
However, our interviews highlighted that users’ actual needs are often more modest than this. Most researchers and start-ups primarily need access to small clusters of GPUs for development work rather than massive parallel computing capability. They might also require more extensive support infrastructure, software environments and skills development.
AI-focused companies typically prefer systems optimised specifically for AI workloads, with architectures built around GPUs and modern software stacks. Experts we spoke to suggested that these projects often need flexible, on-demand access rather than having to bid for time on large shared systems. For smaller companies and start-ups, access to even modest GPU resources can be transformative, especially given the cost of accessing cloud resources at market rates.
Interviewees indicated that government agencies and public-sector organisations have particular needs which are different to those of other user groups. They often need compute for specific applied use cases rather than pure research, which often creates requirements around security and data governance.
Some interviewees also mentioned that when governments attempt to procure hardware explicitly for AI, they narrow down the list of companies from which they can procure and in the process reduce their own bargaining power.
Even if AI development is one of the goals that a given government is interested in, designing narrow procurement policies exclusively around this goal can send counterproductive signals to the market and drive up the price of AI hardware.
Models of public compute provision
The mechanism through which public compute is delivered varies considerably across jurisdictions. The following key approaches have emerged from our study:
- Direct public provision: the state procures, owns and operates compute infrastructure directly.
- Arms-length provision: the state delivers compute through independent entities that may be publicly funded but operate autonomously.
- Public–private partnerships: the state collaborates with private firms to provide compute services.
- Private provision with public funding: private entities provide resources with public subsidies.
- Direct subsidies or vouchers: the state provides financial support for organisations to access private compute.
Figure 1: Models of public compute provision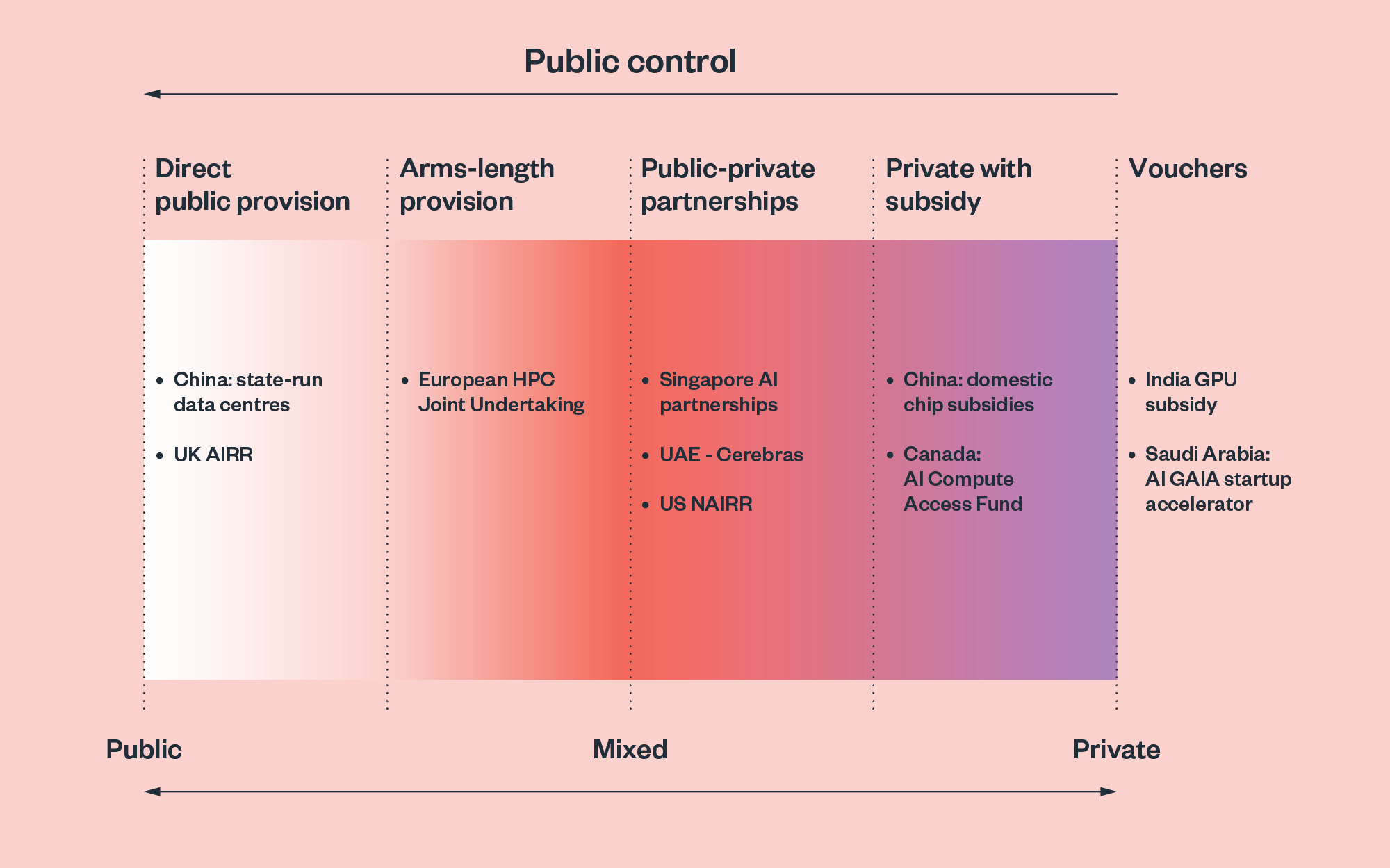
Most existing initiatives follow hybrid models that leverage private-sector expertise and resources while maintaining public oversight. This reflects practical considerations around cost and operational expertise, but risks relinquishing public control, subsidising large players and jeopardising alignment with policy objectives.
Access and use conditions
Access policies often reflect broader strategic priorities around innovation, security and economic development. Common conditions include:
- development practices and safety requirements
- licensing and commercial model restrictions
- the particular legal status of the recipient, such as being registered as a non-profit
- requirements around open publication of research
- commitments to public benefit applications, which can be both broadly or narrowly specified.
Our interviews indicated that in most jurisdictions the introduction of usage conditions for organisations accessing public compute resources is being actively explored, with open publication and the adoption of particular licences the most common requirement. At present, profit sharing or equity arrangements and restrictions on downstream commercialisation are relatively underutilised, reflecting the tendency of these initiatives to focus on basic research rather than downstream commercial applications.
Supporting resources
Existing public compute initiatives are often conceived holistically – not just as providers of raw compute, but as comprehensive programmes that build capacity, communities and infrastructure for advancing AI research and development.
One crucial complementary offering is technical expertise and support services. Our interviews highlighted that many researchers and organisations lack the specialised skills needed to effectively utilise high-performance computing (HPC) resources, requiring assistance with software environments, training and troubleshooting. As such, public initiatives frequently provide training and upskilling programmes, staff for provisioning support with technical issues, and assistance with networking and configuration. Software and database maintenance is a particularly under-supported area in this regard.
Ecosystem building is another focus of many public compute initiatives, which aim not only to provide compute but to catalyse broader innovation ecosystems. For instance, some initiatives have created platforms for equipment and resource sharing between data centres, knowledge exchange between institutions, and the formation of research communities around specific technologies or applications. This can include trying to catalyse communities of practice: the Edinburgh Parallel Computing Centre in the UK explicitly aims to foster collaboration between academic and industry partners, while the US’s NAIRR pilot includes educational and outreach programmes specifically targeted at smaller and lesser-known institutions.
Finally, public compute initiatives often provide access to further resources. These include access to valuable datasets, specialised software tools and frameworks, storage infrastructure and high-speed networking capabilities. Like the US’s NAIRR, they can also facilitate direct, subsidised access to private-sector AI models.
Cost and funding models
Costs of public compute initiatives vary enormously depending on the scope and delivery model. They can typically be funded through direct public investment, public-private agreements or other approaches such as user charging.
Traditional supercomputing facilities can require investments in the hundreds of millions or into the billions of dollars, while targeted subsidy programmes might operate at much smaller scales. The UK’s paused investment in an exascale supercomputer, estimated at around £900 million over five to six years, illustrates both the scale of investment required and the political challenges of securing long-term funding commitments.[31]
Several key factors drive this variance in costs. Large, centralised facilities like exascale systems (the current state-of-the-art systems, referring to computers that can calculate 10^18 FLOPS per second) represent the highest-cost approach. Smaller, distributed systems or GPU clusters can be built for tens to hundreds of millions. The choice between CPU-only versus hybrid GPU architectures significantly impacts costs, with high demand for GPUs making the latter more costly. Ongoing operational expenses are influenced by power consumption and energy efficiency, which can vary significantly by geographical location. In some jurisdictions, initiatives like China’s ‘Eastern Data, Western Computing’ and New York State’s Empire AI aim to reduce these costs by locating data centres in areas with abundant energy or water resources.[32]
Cost and funding in public compute resources often reflect national political priorities to fund certain scientific research areas that require these resources.
Public compute initiatives employ diverse funding models that reflect both strategic priorities and institutional constraints across different jurisdictions. At their core, most rely on significant direct government funding, typically channelled through research councils or science and technology ministries. These allocations often require major spending review decisions and can become subject to political challenge, as seen in the UK.
However, pure central government funding represents just one approach. The EuroHPC Joint Undertaking demonstrates a successful multi-level government collaboration, splitting funding 50/50 between the EU and member states. This model allows individual nations to maintain control over their share while achieving the scale benefits of pooled investment. In China, some public compute initiatives are funded and run by local governments.
Public-private partnerships represent another significant model, exemplified by the US’s NAIRR pilot which combined $30 million in federal funding with $80 million in private sector contributions. These partnerships often involve in-kind resources or direct investment from industry partners, including cloud credits, hardware donations or operational support. While this approach helps scale access, it raises important questions about control and value capture, which are discussed further below.
Design options for public compute
This section details four broad families of public compute initiatives that have emerged from our study of such initiatives across several countries. Governments have tried to design and modify public compute programmes with a view to optimising for selected policy objectives.
Direct provision (generalist) | Direct provision (AI-focused) | Decentralised provision | Market-based provision | |
Description | Large-scale, general-purpose infrastructure aimed at servicing multiple use cases – from academic research to public-sector projects – through flexible architecture. Typically involves significant capital investment in facilities housed within national laboratories or research institutions.
|
Infrastructure specifically designed for computationally intensive AI workloads such as ‘frontier’ LLM development, multi-modal and multi-agent systems.
These initiatives are typically GPU-centric and optimised for AI workloads, with more flexible access patterns and integrated AI-specific support services. |
The use of public resources to facilitate networks of smaller facilities distributed across regions. These facilities can themselves be publicly- or privately-owned.
|
The use of public funds to provide financial incentives, vouchers or subsidies to help users access compute from existing commercial providers.
|
Examples | US Department of Energy supercomputers; European High-Performance Computing (EuroHPC) Joint Undertaking | AI Research Resource (UK); National AI Research Resource (US) | Open Cloud Compute (India); China’s network of municipally-owned datacentres | Compute vouchers provided as part of India’s IndiaAI mission |
Effective for | 1. Supporting scientific research
2. Supporting small and medium enterprises 3. Digital sovereignty for critical uses like military or strategic communications |
1. Supporting AI research, including AI safety research
2. Supporting the domestic AI industry 3. AI sovereignty 4. Market shaping |
1. Market shaping
2. Supporting the domestic compute industry |
1. Supporting the domestic AI industry
2. Supporting small and medium AI enterprises 3. Supporting scientific research |
Potential drawbacks | Risk of spreading investment too thinly across different types of compute.
Usually takes the form of large projects, which present coordination and hardware specification difficulties. |
Risk of entrenching monopolies when not paired with market shaping measures. | Large scale versions have yet to be tried: it is not clear that decentralisation can work at national scales with very large investments.
Ignores chip design and manufacturing layers. |
Risk of simply subsidising incumbent companies.
Does not tackle dependencies in the compute supply chain and cannot on its own contribute to digital or AI sovereignty. |
Direct provision (generalist)
The provision of public compute through large-scale, general-purpose infrastructure represents one of the most established approaches to expanding compute access. These initiatives typically involve significant capital investment in facilities aimed at serving both broad research communities and strategic national interests. The US Department of Energy’s (DOE) supercomputing programme exemplifies this model, with facilities designed to support multiple use cases – from academic research to public-sector projects – through flexible architecture housed within national laboratories or research institutions.
These investments require substantial long-term operational planning to create, run and maintain, often on five- to ten-year horizons, and focus on building general capability rather than supporting specific applications. The DOE’s approach illustrates this well, aiming to support a remarkably diverse range of workloads, from weather forecasting to COVID-19 research.
The advantages of this approach are significant. Large facilities can leverage economies of scale in both procurement and operations. The DOE, for instance, claims to have achieved a 400 per cent improvement in energy efficiency compared to industry expectations. Their general-purpose nature also provides strategic flexibility to adapt to emerging needs, with hybrid chip architectures proving crucial for supporting both traditional high-performance computing (HPC) workloads and newer AI applications. These facilities also play a vital role in developing the broader research community, fostering collaboration and building essential skills. The EU’s HPC Joint Undertaking initiative similarly shows the advantage of scale, as a project designed to overcome the challenges of running multiple small computing clusters across the EU, and to instead build large or connected clusters by pooling resources.
However, generalist provision faces several key challenges. Poor specification can severely limit an initiative’s aspiration to general use. The UK’s ARCHER2 system, built entirely on CPU architecture, is now seen by some as having made the ‘wrong bet’ on hardware, because such architectures are poorly-suited for AI research and the initiative therefore has a more limited range of applications than intended. Large projects also require complex stakeholder coordination and present persistent challenges around the prevalence of legacy code, with many users running outdated software that limits efficiency.
These challenges point to several key policy implications. Success requires long-term strategic planning and commitment. Interviewees suggested that the UK in particular has suffered from having to repeatedly make the case for new national services rather than working from a comprehensive long-term plan, although the commitment in the AI Opportunities Action Plan to set out a new long-term plan aims to remedy this. Others, like the EU, continue to upgrade or change the hardware they provide through existing systems.
Governance structures need to carefully balance the flexibility required to allow research autonomy with long-term government strategic priorities.
We address this challenge in further detail below. Nevertheless, generalist provision remains important in the face of uncertainty about the utility of large language models or other similar AI models to scientific research. Generalist provision can be more flexible and allow for pivots towards or away from AI as an investment strategy.
Direct provision (AI-focused)
In contrast to generalist provision, a new model of public compute specifically designed for computationally-intensive AI workloads such as ‘frontier’ LLM development, multi-modal and multi-agent systems has emerged. These initiatives differ markedly in their architecture, access models and strategic objectives. They typically feature GPUs, optimisation for AI workloads, more flexible access patterns and integrated AI-specific support services.
The UK’s Isambard-AI project exemplifies this approach, prioritising flexibility while maintaining a clear focus on AI model training and inference rather than general-purpose computing for simulations and modelling. Similarly, the US’s National AI Research Resource pilot demonstrates how AI-focused provision often involves novel public-private collaboration models, bringing together government partners with technology companies.
Key considerations for AI-focused provision include carefully calibrating scale and architecture to varying use cases. While some projects require massive compute resources, many researchers begin with limited datasets and need only a few GPUs. Access models must therefore be flexible enough to accommodate different usage patterns. Perhaps most crucially, these initiatives require substantial technical expertise.
These initiatives also face distinct challenges. There is significant uncertainty around demand, with decisions about expenditure and procurement resting on judgement rather than reliable forecasts. Technical integration poses another challenge, particularly when working with diverse hardware – a challenge for teams looking to network different types of chips effectively, or even when trying to network a large number of similar chips. Government teams and beneficiaries do not always have the requisite specialised software skills for using a large number of GPUs. Many governments currently rely on private-sector hardware providers for training in compute software paradigms, a dependency that is open to exploitation by incumbent companies. Perhaps most fundamentally, there is a risk of public investment primarily benefitting large tech companies, who seek to use public systems to train and run models while retaining all of the value generated. In addition, the AI hardware market is concentrated and public procurement or subsidisation of this hardware can mean the funnelling of public funds into already highly valued and monopolistic corporations.
Based on our interviews and a review of policy documents, AI-focused provision seems to be based on an assumption that advanced foundation models, broadly understood, can lead to scientific developments and breakthroughs.
The companies that control AI model production today may not prioritise the type of research that leads to scientific breakthroughs, and there is a role for governments to fund researchers and smaller businesses that might want to explore this research with AI models.
While this assumption may not be entirely wrong, it is significant. Google DeepMind’s breakthroughs usually serve as the evidence base for this assumption, but scepticism has been raised about their value.[33] In any case, the success of these projects often requires training systems in large part on simulation data (such as the UK’s Met Office simulations for weather forecasting) which use different hardware. Prioritising AI-focused compute at the expense of other types of research could result in this pool of training data no longer being available – illustrating the interconnectedness of the research ecosystem.
These challenges suggest several key policy implications. Initiatives need to balance broad access with strategic priorities. Success also requires building supporting infrastructure, including chip development capabilities, as highlighted by the investments of the EuroHPC Joint Undertaking. Finally, robust accountability mechanisms are needed to ensure public benefit from these investments.
Decentralised provision
Decentralised provision offers an alternative to centralised national infrastructure, involving networks of smaller facilities distributed across regions, which could themselves be publicly- or privately-owned. The contrasting experiences of China and India illuminate both the potential and limitations of this approach. While both countries pursue decentralisation, they do so quite differently: China adopts a hybrid approach combining municipal initiatives with central coordination, while some in India pursue a more purely decentralised model focused on micro data centres and edge computing.
China’s model includes both company-led and government-led projects, with novel coordination mechanisms like equipment exchange platforms.[34] India’s Open Cloud Compute (OCC) model, a platform for accessing micro data centres run by private entities, relies more heavily on opportune public-private contracts without strong central coordination.[35]
Both approaches face some challenges. There is an inherent tension between broad access and strategic capabilities: dispersed micro data centres may not provide sufficient strategic advantages in a race towards global competitiveness if scaling still proves to be a driver of model capabilities. Infrastructure requirements pose another major hurdle, particularly in India where fundamental challenges remain around networking thousands of miles of territory. Both countries also grapple with technical integration issues, particularly around networking diverse hardware components.
The experience in China suggests several success factors for decentralised provision. Significant local state capacity appears crucial. Novel coordination mechanisms can enhance effectiveness, as demonstrated by China’s equipment exchange platform. Supporting infrastructure and technical capabilities at the local level are also essential.
In our view, decentralised provision is a useful corollary to other kinds of public compute provision and enables compute policies related to market structure. Decentralised provision can allow for more public-oriented and diversified innovation to flourish when supported by other policies.
Market-based provision
Market-based provision represents yet another approach, one which uses financial incentives, vouchers or subsidies rather than direct infrastructure investment. This model typically focuses on demand-side interventions and often involves providing subsidies to existing commercial providers. Implementation can take various forms, from governments providing cloud credits[36] and voucher schemes to public-private partnerships. India’s compute provision under the IndiaAI mission is part of this category, as it provides subsidies for the use of a small subset of AI chips to approved entities. These entities, usually researchers, non-profits or government organisations, can choose the kinds of chips or cloud access they require, and then apply for a subsidy.
This approach can help to expand access while leveraging existing infrastructure, and creative procurement strategies can yield significant savings while not requiring governments to make very specific decisions on the kinds of hardware to procure. A further benefit is that some risks under this model continue to be borne by the private and research sectors, with less exposure for public entities.
However, market-based provision faces distinct challenges. It risks reinforcing existing market concentration and affords public institutions less control over the infrastructure and downstream applications. According to our interviewees, some market-based provision approaches can end up in a form where governments mediate and inefficiently or unnecessarily subsidise access between large domestic companies seeking to train AI models and large foreign companies able to supply advanced AI hardware. Market-based provision can also risk the government over-specifying the type of hardware that is eligible, as well as the types of use the hardware can be put towards.
Recommendations for the future development of public compute policies
Despite the diversity of public compute initiatives, we can identify several common challenges across jurisdictions that require further attention from policymakers.
Avoiding value capture
The substantial public investment required for compute infrastructure raises important questions about how to balance broad access with creating returns to the public purse and avoiding capture by a few private beneficiaries. While existing public compute initiatives primarily aim to serve the research community, their procurement decisions can have significant market-shaping effects on suppliers and users.
Through strategic procurement, these initiatives can potentially advance broader policy goals such as developing domestic supply chains and promoting energy efficient technologies. However, policymakers need to be realistic about what can be achieved through public compute projects alone. The global nature of semiconductor supply chains, which are dominated by a few key players like Nvidia, means that for most jurisdictions the goal of ‘onshoring’ production will likely be a near impossibility in the short to medium term.
One key challenge is value capture: the risk of public investment primarily benefitting private interests, either through the direct use of facilities or through the commercialisation of research outputs. The risk of value capture can be mitigated through careful policy design around access and licensing conditions for research and products developed with the support of public compute initiatives. Some jurisdictions are exploring requirements for open publication of results or commitments to applications for public benefit. However, specific mechanisms remain underdeveloped and many of the appropriate levers will lie with other actors or institutions, such as research funding agencies, universities and regulatory bodies.
Recommendations
Recommendation 1: Insulate public compute strategies from industry lobbying
While industry engagement is valuable for understanding technical developments and user needs, public compute strategies must ultimately serve broader societal interests. Governments should establish robust governance mechanisms for public compute initiatives that can resist pressure from powerful incumbents while remaining responsive to legitimate stakeholder input.
One approach is to create independent advisory bodies for managing public compute initiatives and allocating resources, with diverse membership from academia, civil society and smaller industry players, not just large tech firms. These bodies should operate with high levels of transparency and clear protocols for managing conflicts of interest.
The experience of other sectors, such as financial regulation, offers useful lessons. For instance, implementing ‘cooling off’ periods before officials can work for regulated entities. Additionally, funding decisions should be based on clear, publicly-stated criteria rather than behind-the-scenes influence. The EU’s experience with European High-Performance Computing Joint Undertaking demonstrates both the importance and challenge of maintaining strategic independence while coordinating with industry partners.
Recommendation 2: Target procurement as part of a holistic industrial strategy for compute
While current market conditions may necessitate working with dominant suppliers, procurement strategies should actively seek to cultivate a more diverse supplier ecosystem over time. This means moving beyond simple cost considerations to consider how procurement can support emerging players and alternative technologies.
For example, public compute facilities could dedicate a portion of their capacity to testing and validating new hardware solutions from smaller firms. Government procurement functions (for example, the Crown Commercial Service in the UK) should also prioritise modular, standards-based approaches that reduce lock-in to particular vendors. However, this must be balanced against the need for interoperability and efficiency: trying to integrate too many different systems can create technical challenges that undermine the facility’s usefulness.
Recommendation 3: Explore conditionality for user access to compute
Public compute resources represent a significant investment of taxpayer funds and should generate clear public benefits. Access models should include carefully designed conditions to ensure this, while avoiding overly restrictive requirements that could deter valuable research and innovation.
For instance, facilities might require users to openly publish research findings and share trained models, while allowing subsequent commercial applications. Requirements around responsible AI practices and safety testing could also be incorporated, with the input of regulators and standards bodies. The US’s National AI Research Resource pilot offers useful lessons here: its emphasis on open science principles provides a starting framework, though implementation details remain to be worked out. The key is striking a balance between ensuring public value and maintaining flexibility for different use cases.
Recommendation 4: Provide adjacent resources to ensure a wide range of organisations can benefit
Simply providing raw compute capacity is insufficient. Many potential users, particularly smaller organisations and research institutions, need additional support from government to effectively utilise these resources. This should include technical assistance with tasks like networking and optimisation, training programmes to build relevant skills, and help navigating access processes.
Additionally, funding for associated costs like data collection, storage and preparation may be necessary. Programmes should also consider providing vouchers or credits to help organisations test and scope compute-intensive projects before making major commitments. The goal should be to lower barriers to entry while building long-term capacity across the ecosystem.
Recommendation 5: Consider complementary interventions to address market concentration
Public compute initiatives alone cannot address the fundamental market dynamics that have led to concentration in AI infrastructure. Governments should pursue parallel regulatory interventions to promote competition and prevent further entrenchment of dominant positions. This could include measures to ensure interoperability between different compute platforms and prevent anti-competitive tying of hardware, software and cloud services.
Drawing on established competition frameworks from other network industries, regulators might require dominant firms to provide fair access to key technologies like CUDA that have become de facto standards. The EU’s Digital Markets Act and the UK’s Digital Markets, Competition and Consumers Act provide potential models, though additional provisions may be needed to address the specific characteristics of AI infrastructure markets.
Structural interventions may also be necessary. These could include restrictions on vertical integration that allows firms to leverage dominance across multiple layers of the AI stack. For instance, regulators might scrutinise arrangements where cloud providers both operate infrastructure and compete with their customers in AI development. While such measures would need careful design to avoid disrupting legitimate efficiency benefits, experience from sectors like telecommunications shows that structural separation can promote innovation while preserving scale economies.
Achieving strategic coherence
The institutional landscape for public compute is increasingly complex, with initiatives operating at municipal, state and federal levels in many jurisdictions including China, the EU and the USA. This creates significant challenges around coordination and strategic alignment, both between different compute projects and with the broader legal and policy framework for AI development and deployment.
The effective use of public compute resources can be constrained by limited access to complementary factors, particularly high-quality data and specialised technical skills. Interviewees told us that there is a particular shortage of expertise in areas like networking and compute software optimisation. While collaboration with industry can provide short-term access to these resources, longer-term solutions may require broader policy interventions. These could include targeted skills initiatives, competition policy to address market concentration and public investment in data infrastructure.
The experience of several jurisdictions suggests that realising the full benefits of public compute investments requires careful attention to these complementary factors. Simply providing hardware access, without addressing wider ecosystem constraints, is unlikely to achieve policy objectives around innovation and competitiveness – but the agencies developing these initiatives do not always have the necessary authority or remit to drive these wider goals.
Recommendations
Recommendation 6: Ensure mechanisms to coordinate on a national level while preserving local autonomy
Governments should assign coordination responsibilities to existing agencies to align public compute initiatives across different levels of government, while preserving the benefits of local or sectoral experimentation and adaptation. These bodies should bring together representatives from federal, state/regional, municipal and special purpose compute projects, along with relevant policy agencies and technical experts.
The experience in China shows how this coordination can work effectively: regional initiatives maintain significant autonomy in implementation while operating within broader national frameworks for technology development.
These mechanisms should focus on sharing best practices, avoiding duplication and identifying opportunities for collaboration, rather than imposing rigid top-down control. An important role can also be played by interoperable standards that allow for public investment in compute across different levels of government, such that users can switch between or jointly use compute made available by both regional and national government organisations.
Recommendation 7: Develop integrated strategies for compute infrastructure and skills
Public compute initiatives need to be embedded within broader strategies that address complementary needs, particularly around skills and data infrastructure. This means moving beyond treating compute provision as a standalone technical challenge to considering the full ecosystem needed for effective utilisation.
Drawing on experience from existing facilities, governments should establish dedicated programmes to build expertise in critical areas like networking and optimisation. These could include specialised training programmes, partnerships with educational institutions, and incentives or even requirements for knowledge transfer from industry. Additionally, skills development should be coordinated across different compute initiatives to build a shared talent pool and create clear career pathways.
Recommendation 8: Coordinate for energy and water requirements
The high energy and water requirements of AI compute mean that efficiencies can be gained through coordination with public providers of energy and water resources, as well as public investments in energy and water infrastructure, particularly infrastructure with a relatively lower climate and environmental footprint. Such long-term coordination can lead to economic savings and progress towards environmental goals.
Public compute initiatives should actively coordinate with clean energy development. This could include co-locating facilities with renewable energy projects, investing in energy storage to enable more flexible operations, and developing smart systems to optimise workload scheduling based on energy availability.
One dynamic to be cautious about is the inadvertent redirection of public energy and water resources away from disadvantaged people and towards speculative AI endeavours.
Balancing flexibility and longevity
Public compute strategies must navigate significant uncertainty about AI’s future development, capabilities and markets. Some jurisdictions have responded by maintaining flexibility in their approach: India, for example, has shifted from direct public provision of compute resources to a subsidy-based model supporting private-sector access.[37]
However, this flexibility can come at a cost. Infrastructure investments typically require stability and predictability to attract private sector engagement and investment. The recent partial cancellation of the UK’s public compute investment illustrates how policy changes can undermine confidence and deter private-sector participation.[38] The backlash from industry and researchers highlights concerns about the UK’s commitment to maintaining internationally competitive research infrastructure,[39] and was swiftly followed by a renewed commitment in the AI Opportunities Action Plan.
Finding the right balance between adaptability and stability presents a key challenge for policymakers. While strategies need to be responsive to technological change and emerging needs, frequent pivots or reversals can undermine the long-term effectiveness of public compute initiatives. By the same token, demand for compute in the long run is difficult to gauge: if the compute needs of leading AI models drop dramatically, then public initiatives run the risk of over-investment in assets that will not be likely to deliver sufficient returns. Proper calibration is therefore essential if public compute initiatives are to deliver for the public.
Recommendations
Recommendation 9: Set long-term targets and funding envelopes, but with flexibility on delivery
Governments should establish formal mechanisms for periodically reviewing and updating public compute strategies. These reviews should examine both technical requirements and broader policy alignment, with clear processes for incorporating findings into future planning.
Reviews might occur on a rolling basis. For instance, conducting detailed technical assessments annually alongside broader strategic reviews every three to five years. This provides a structured way to maintain strategic continuity while adapting to change. The process should include diverse stakeholder input while maintaining independence from industry capture.
Governments should also set out decade-long funding envelopes and capability targets, providing the certainty needed for strategic planning and recruitment. However, procurement and spending decisions should remain adaptable. The UK’s experience with ARCHER2 illustrates the risks of rigid hardware specifications: the purely CPU-based architecture quickly became suboptimal as AI workloads grew in importance. A better approach would be to establish broad performance and accessibility goals while preserving flexibility on technical implementation. This could include options for phased procurement or modular infrastructure that can be updated as needs evolve. The model being pioneered at facilities like Bristol’s Isambard-AI, which uses modular data centres that can be efficiently upgraded, offers a promising template.
Recommendation 10: Build modular software infrastructure alongside hardware
While hardware flexibility is crucial, policymakers should pay equal attention to creating adaptable software infrastructure. Public compute facilities should invest in developing modular and interoperable software stacks that can accommodate different hardware configurations and evolving user needs.
Through our interviews, we have learned that the experience of facilities like the Edinburgh Parallel Computing Centre (EPCC) shows that software dependencies often create more long-term constraints than hardware choices. Facilities should prioritise open standards and interfaces that allow components to be updated independently. This approach helps future-proof investments while reducing dependency on the ecosystems of particular vendors, which can risk making public compute investments into a subsidy for incumbent players. Interviews also revealed that the US Department of Energy’s experience with scientific computing offers valuable lessons here: its emphasis on portable software has enabled smooth transitions across multiple hardware generations.
Squaring compute investments with environmental goals
A final challenge relates to the need for these initiatives to square strategic objectives on AI with the significant environmental costs of building compute infrastructure. This may conflict with national climate and environmental goals and, in some cases, legal obligations. Data centre facilities have significant energy demands, which are estimated by the IEA to account for around 1 –1.5 per cent of global electricity use and 1 per cent of energy-related greenhouse gas emissions.
Although global electricity consumption for data centres has grown modestly, countries where data centres are concentrated, like Ireland, have seen data centre electricity usage triple between 2015 and 2023, accounting for 21 per cent of the country’s total electricity consumption by 2023.[40] EirGrid, Ireland’s state-owned electricity transmission operator, predicts that due to significant growth in the data centre market in Ireland, data centre energy usage could account for 28 per cent of national demand by 2031.[41] Ireland’s Environmental Protection Agency has stated that it will miss its 2030 carbon reduction targets by a ‘significant margin’.[42]
Compute architecture can also consume water at significant rates to assist in evaporative cooling to prevent servers from overheating and, in some cases, humidity regulation. In Microsoft’s latest sustainability report, it acknowledges that its annual water consumption has increased by 187 per cent between 2020 and 2023 to 7.8 million cubic metres (km3).[43] In comparison, Loch Ness in Scotland has a volume of 7.4 km3.[44]
Investments in public compute risk intensifying these dynamics if they are not coupled with measures to reduce the climate and environmental impact of data centres and their associated infrastructure. Strategies for this are under active consideration by policymakers across jurisdictions. One prominent suggestion is the use of selective procurement to incentivise energy and water efficiency, but it is likely that more robust action will be necessary to ensure that data centre buildouts do not compromise climate and environmental obligations.
Recommendations
Recommendation 11: Review the impact of AI on data centre demand, including the role of public compute initiatives
Policymakers need a clearer understanding of how AI development is affecting data centre demand and energy consumption. While existing projections suggest significant growth, they often fail to account for the unique characteristics of AI workloads or the potential role of public compute facilities.
Governments should commission detailed assessments that examine both direct energy use and broader system effects. These reviews should consider how public compute initiatives might influence overall demand – for instance, whether consolidated public facilities could be more efficient than dispersed private alternatives. The experience of facilities like the EPCC shows that strategic choices around location and infrastructure can significantly impact environmental outcomes. Reviews should also examine the relationship between compute access models and energy use patterns, considering whether certain allocation approaches might encourage more efficient resource utilisation.
Current approaches to measuring compute facilities’ environmental impact often focus too narrowly on direct energy consumption. One aim of any review should therefore be to develop more comprehensive frameworks that consider full lifecycle impacts, including the manufacturing and disposal of equipment, land acquisition challenges, noise, water usage and broader system effects. These metrics should inform both facility design and operational decisions. Drawing on emerging best practices in environmental accounting, facilities should be required to report against standardised impact measures. This data can then guide future investment decisions and help identify opportunities for improvement.
Recommendation 12: Require environmental commitments from suppliers and users
Public compute initiatives should embed sustainability requirements throughout their operations. For suppliers, this means setting clear standards for energy efficiency and requiring detailed reporting on environmental performance. Procurement processes should evaluate lifecycle emissions, not just operational energy use, and could include requirements for equipment longevity and repairability.
For users, facilities should implement policies that encourage efficient resource use – for instance, differential pricing or allocation priorities based on workload efficiency. Drawing on experience from other sectors, facilities might require users to report on energy consumption and demonstrate efforts to optimise their workloads.
The modular data centre approach being pioneered by Bristol’s Isambard-AI shows how infrastructure choices can support both environmental and operational goals – for instance, by enabling heat recovery for district heating systems and facilitating equipment upgrades without wholesale replacement. These requirements must be carefully designed to avoid creating unnecessary barriers to access, particularly for smaller organisations or novel applications.
Conclusion
Public compute has emerged as a critical frontier in AI policy. As this report has shown, there is no one-size-fits-all approach to getting public compute provision ‘right’. Different jurisdictions have adopted varying strategies based on their existing capabilities, policy frameworks and strategic objectives.
What unites successful approaches is their recognition that public compute policy must go beyond simply providing hardware access. Effective initiatives require careful attention to supporting infrastructure, skills development and market dynamics. They must also balance competing priorities: between strategic autonomy and international collaboration; between supporting frontier LLM development and other types of computational research; and between advancing AI capabilities and meeting environmental obligations.
The recommendations in this report outline concrete steps policymakers can take to navigate these tensions. From establishing robust governance mechanisms that resist industry capture, to developing integrated strategies for compute infrastructure and skills, these recommendations provide a framework for designing public compute initiatives that genuinely serve the public interest.
As AI continues to reshape economies and societies, the stakes for getting public compute policy right are high. Without careful policy design, public investments risk primarily benefitting incumbent players and entrenching existing market concentration. However, when thoughtfully implemented with appropriate conditions and complementary policies, public compute initiatives can help democratise access to AI development, support public interest research and ensure a more equitable distribution of AI’s benefits.
The challenge for policymakers is not just to provide compute resources, but to do so in ways that actively shape markets, support diverse innovation and advance broader societal goals. Success requires moving beyond seeing public compute as merely an infrastructure for supporting technical research to understanding it as a key lever for influencing how AI develops and who it serves.
Acknowledgements
This report was lead authored by Matt Davies and Jai Vipra. The authors are grateful to Michael Birtwistle, Catherine Gregory, Brandon Jackson, Elliot Jones, Nik Marda, Joshua Pike, Elizabeth Seger, Eleanor Shearer, Bhargav Srinivasa Desikan, Andrew Strait and Max von Thun for their comments and substantive contributions.
The authors would also like to thank the following individuals for agreeing to take part in the interviews which this report draws on:
- Katerina Antypas, National Science Foundation (USA)
- Jeffrey Ding, George Washington University (USA)
- Graham Dobbs, Toronto Metropolitan University (Canada)
- Travis Hoppe, White House Office of Science and Technology Policy (USA)
- Ben Johnson, University of Strathclyde (UK)
- Tanvi Lall, People + AI (India)
- Simon McIntosh Smith, University of Bristol (UK)
- Amlan Mohanty, independent (India)
- Teri Olle, Economic Security Project California (UK)
- Mark Parsons, University of Edinburgh (UK)
- Nitarshan Rajkumar, University of Cambridge (UK)
- Farhat Raza, Department for Science, Innovation and Technology (UK)
- Satya S. Sahu, independent (India)
- Julia Stoyanovich, New York University (USA)
- Stuart Styron, Office of Representative Anna Eshoo (USA)
- Josephine Wood, EuroHPC Joint Undertaking (EU)
- Charles Yang, Department of Energy (USA)
Finally, we would like to extend our gratitude to those who took part in expert roundtables as we planned and developed this work:
- Haydn Belfield, Leverhulme Centre for the Future of Intelligence
- Carsten Jung, Institute for Public Policy Research
- Mina Mohammadi, Mozilla Foundation
- Vidushi Marda, AI Collaborative
- Sarah Myers West, AI Now Institute
- Elizabeth Seger, Demos
- Eleanor Shearer, Common Wealth
- Mary Towers, Trades Union Congress
- Max von Thun, Open Markets Institute
Any mistakes or errors made in this work are those of the authors alone and should not be attributed to any of the individuals listed above.
Appendix – Case studies
Public compute policies are heavily influenced and circumscribed by exogenous factors, including the international environment, domestic market dynamics and the relative strengths of each country. Countries often tailor their public compute strategies based on their existing policy frameworks and areas of comparative advantage. This means that national public compute policies reflect broader geopolitical, economic and technological conditions.
The following case studies show how different jurisdictions are approaching the provision of public compute, and indicate some of the relevant ‘shaping factors’. For each country or bloc, we have listed key features of public provision, as well as factors shaping the continued development of strategy.
China
China’s approach to public compute is characterised by significant decentralisation and multiple overlapping initiatives at different levels of government:
- Implementation of state and municipal compute initiatives, including direct provision of compute resources through state-run data centres and regional AI labs.
- City-level compute voucher schemes offering 40–50 per cent cost reductions for AI start-ups and research.
- Novel coordination mechanisms like government-supported equipment exchange platforms to address supply chain bottlenecks.
- Strategic ‘Eastern Data, Western Computing’ initiative to build compute centres in western provinces to leverage cheaper energy costs.
- State-level subsidies increasingly focused on equipment and materials rather than just chip design/fabrication.
China’s approach is shaped by the following:
- International context: responding to export controls by building domestic capabilities across the full computing stack while maintaining access to global technology where possible.
- Domestic priorities: balancing support for large tech companies who can stockpile chips with smaller players who face greater constraints under export controls.
- Policymaking approach: significant provincial/municipal autonomy in implementation within broader national strategic frameworks, enabling local experimentation while maintaining central coordination.
- Technical challenges: growing focus on developing software stacks for domestic hardware and building skills for networking compute systems.
EU
The European High-Performance Computing (EuroHPC) Joint Undertaking was established in 2018 and is a collaborative initiative involving the EU, 32 European countries and three private partners. Funded 50 per cent by participating states and 50 per cent by the EU budget, the EuroHPC Joint Undertaking aims to develop and maintain a world-class supercomputing ecosystem in Europe.
Key features relevant to AI include the following:
- Acquiring, upgrading and operating AI-dedicated supercomputers.
- Providing open access to these resources for both public and private entities, with free access for research projects. This is limited to organisations in the EU or participating states.
- New focus on AI model training in strategic sectors like healthcare, climate change, robotics and autonomous driving, redeploying existing allocated resources to support this expansion.
- Emphasising support for start-ups and medium-sized enterprises, with special access conditions for these entities.
- Developing European hardware capabilities through initiatives like the European Processor Initiative.
The EU approach is shaped by several key factors:
- International context: positioning Europe as a competitive force in global AI development.
- Policymaking comfort zone: leveraging existing multi-national collaborative frameworks (for example, Horizon Europe).
- Adaptation challenges: balancing traditional high-performance computing (HPC) users with new AI-focused users, while prioritising strategic sectors and smaller enterprises.
France
France’s approach to public compute is characterised by significant national investment, strategic partnerships and a focus on digital sovereignty:
- Major investments in HPC infrastructure, including GENCI, to support scientific research and industrial innovation.
- Implementation of a National AI Strategy, launched in 2018, to increase access to compute resources for AI development and research, and support for AI-focused institutes like Inria (Institute for Research in Computer Science and Automation) to support compute-heavy AI and machine learning projects.
- Continued participation in European initiatives like the EuroHPC Joint Undertaking to build and operate world-class supercomputing infrastructure.
- Emphasis on digital sovereignty through projects like Gaia-X, ensuring critical digital infrastructure remains under national or European control.
- Direct funding and incentives through initiatives like France Relance to boost investments in computing infrastructure, AI and quantum computing.
France’s approach is shaped by:
- International context: positioning France as a leader in AI and HPC while maintaining digital sovereignty.
- Domestic priorities: aligning compute policies with broader industrial strategy to support key sectors like healthcare, aerospace and automotives.
- Policymaking approach: balancing national investments with public-private partnerships and participation in EU-wide initiatives.
India
India is pivoting towards a more flexible, market-focused strategy:
- A planned investment of approximately Rs. 4,568 crores (£417,000) over five years to partially subsidise GPU procurement costs for Indian companies.
- Companies benefitting from the subsidy will have the freedom to choose their preferred chips while availing of the subsidy.
- Government oversight to prevent misuse or resale of subsidised compute resources.
- Implementation of an empanelment (selection) process for compute providers to join the public initiative, with current requirements favouring larger, established players.
- The Open Cloud Compute (OCC) project, a non-governmental initiative which aims to create an alternative supply pool of compute resources, working with smaller providers.
The overall strategy represents a shift from an earlier plan to build a single 10,000-GPU cluster through a public-private partnership.
India’s approach is shaped by several key factors:
- Policymaking comfort zone: a preference for subsidising purchases rather than direct provision.
- Domestic industry: India’s start-up, university and industrial ecosystem is well-developed in some parts of the AI value chain and can benefit from these policies.
- Research gaps: lack of comprehensive data on current compute capacity and future requirements, complicating policymaking.
- Long-term aims: growing interest in developing micro and edge data centres across India, and in green data centre initiatives, with some Indian companies attracting international attention for environmentally friendly designs.
- Complementary policies: India has announced production- and design-linked incentive schemes to encourage domestic design and the fabrication of advanced semiconductors. So far, it has found success in attracting assembly, testing and packaging activities and memory chip designers.
UK
The UK’s approach to public compute is characterised by a combination of direct investment, public-private partnerships and strategic initiatives aimed at supporting AI research, innovation and scientific advancement:
- Investment in HPC facilities like ARCHER2 to support advanced scientific research across various sectors.
- Implementation of a National AI Strategy emphasising widespread access to computing resources for AI research and development.
- Establishment of an AI Research Resource (AIRR) to provide public access to computing infrastructure, software and datasets for AI research.
- Collaboration with private sector companies to enable access to cloud-based compute resources and to foster innovation.
- Direct investment and targeted subsidies through bodies like UK Research and Innovation (UKRI) to support specific industries and research areas.
The UK approach is shaped by the following:
- International context: positioning the UK as a leader in AI and scientific research on the global stage.
- Domestic priorities: focusing on key sectors such as climate science, energy and biosciences.
- Policymaking approach: political churn has led to a lack of long-term clarity and fiscal constraints mean relatively small investment (in global terms).
USA
The USA’s approach to public compute is characterised by a mixture of government-led initiatives, public-private partnerships and regulatory frameworks aimed at advancing access to HPC and AI resources:
- The National AI Research Resource (NAIRR) proposal to provide researchers and academic institutions with access to AI resources, including compute infrastructure, datasets and software.
- Existing government HPC initiatives through national laboratories, with the Department of Energy operating the world’s two largest supercomputers (Aurora and Frontier) and proposing new AI-focused investments in the form of the Frontiers in Artificial Intelligence for Science, Security and Technology (FASST) programme.
- Public-private partnerships facilitating access to cloud computing resources and promoting AI and machine learning innovation.
- Diverse funding mechanisms, including direct state funding, public-private partnerships, subsidies and grants to support access to computing resources.
The US approach is shaped by the following:
- International context: desire to maintain AI leadership and technological innovation on the global stage, and improve the position of US companies in the hardware supply chain.
- Domestic priorities: emphasis on pushing the frontiers of hardware, and on supporting research and innovation in key sectors like AI, healthcare and climate science.
- Policymaking approach: balancing government initiatives with private sector collaboration and market-driven solutions.
- Inter-agency dynamics: including tension between different agencies’ roles in AI and compute initiatives.
Footnotes
[1] The Top500 list ranks supercomputers around the world according to speed on the LINPACK benchmark. ‘Top500 The List’ <https://www.top500.org/> accessed 5 February 2025
[2] See for instance, ‘Public AI’ (Mozilla Foundation, 30 September 2024) <https://foundation.mozilla.org/en/research/library/public-ai/>; ‘Public AI’ (Metagov) <https://metagov.org/projects/public-ai>; Public AI Network <https://publicai.network/>accessed 10 January 2025. For a discussion of the ‘AI stack’, see ‘What is a foundation model?’ (Ada Lovelace Institute, 17 July 2023) <https://www.adalovelaceinstitute.org/resource/foundation-models-explainer/> accessed 10 January 2025.
[3] Vili Lehdonvirta, Boxi Wu and Zoe Hawkins, ‘Weaponized interdependence in a bipolar world: How economic forces and security interests shape the global reach of U.S. and Chinese cloud data centres’ (accepted to Review of International Political Economy)
(15 November2024). <https://ssrn.com/abstract=4670764> accessed 10 January 2025; and Elizabeth Seger and David Lawrence, ‘GB Cloud: Building the UK’s Compute Capacity’ (Demos, 20 May 2024) <https://demos.co.uk/research/gb-cloud-building-the-uks-compute-capacity/> accessed 10 January 2025.
[4] Eleanor Shearer, Matt Davies and Mathew Lawrence, ‘The Role of Public Compute’ (Ada Lovelace Institute, 24 April 2024) <https://www.adalovelaceinstitute.org/blog/the-role-of-public-compute/> accessed 31 October 2024.
[5] Common Wealth <https://www.common-wealth.org/> accessed 31 October 2024.
[6] Jai Vipra and Sarah Myers West, ‘Computational Power and AI’ (AI Now Institute, 27 September 2023) <https://ainowinstitute.org/publication/policy/compute-and-ai> accessed 31 October 2024.
[7] ‘Public AI’ (Mozilla Foundation) <https://foundation.mozilla.org/en/research/library/public-ai/> accessed 31 October 2024.
[8] ‘Public AI: Infrastructure for the Common Good’ (Public AI) <https://publicai.network/whitepaper/> accessed 5 February 2025.
[9] Ibid.
[10] Eleanor Shearer, ‘Take Back the Future: Democratising Power over AI’ (Common Wealth, 11 September 2024) <https://www.common-wealth.org/publications/take-back-the-future-democratising-power-over-ai> accessed 10 January 2025.
[11] Eric Gilliam, ‘ILLIAC IV and the Connection Machine: DARPA’s varied approaches to developing early parallel computers‘ (Good Science Project, 3 November 2023) <https://goodscienceproject.org/articles/illiac-iv-and-the-connection-machine-darpas-varied-approaches-to-developing-early-parallel-computers/> accessed 5 February 2025.
[12] ‘DOE Explains…Exascale Computing’ (U.S. Department of Energy) <https://www.energy.gov/science/doe-explainsexascale-computing> accessed 5 February 2025.
[13] ‘Introduction – Partnership for Advanced Computing in Europe’ (PRACE) <https://prace-ri.eu/prace-archive/about/introduction/>; and ‘Discover EuroHPC JU’ (EuroHPC JU) <https://eurohpc-ju.europa.eu/about/discover-eurohpc-ju_en> accessed 5 February 2025.
[14] ‘ARCHER2’ (EPCC) <https://www.epcc.ed.ac.uk/hpc-services/archer2> accessed 5 February 2025.
[15] Elliot Jones, ‘What is a foundation model?’ (Ada Lovelace Institute, 17 July 2023) <https://www.adalovelaceinstitute.org/resource/foundation-models-explainer/> accessed 5 February 2025.
[16] Madhumita Murgia, ‘Transformers: the Google scientists who pioneered an AI revolution’ (Financial Times, 23 July 2023) <https://www.ft.com/content/37bb01af-ee46-4483-982f-ef3921436a50> accessed 5 February 2025.
[17] ‘Announcing Epoch AI’s Updated Parameter, Compute and Data Trends Database’ (Epoch AI, 23 October 2023) <https://epoch.ai/blog/announcing-updated-pcd-database> accessed 5 February 2025.
[18] Eleanor Shearer, Matt Davies and Mathew Lawrence, ‘The Role of Public Compute’ (Ada Lovelace Institute, 24 April 2024) <https://www.adalovelaceinstitute.org/blog/the-role-of-public-compute/> accessed 31 October 2024.
[19] David Gray Widder, Meredith Whittaker and Sarah Myers West, ‘Why ‘open’ AI systems are actually closed, and why this matters’ (2024) 635 Nature <https://www.nature.com/articles/s41586-024-08141-1> accessed 5 February 2025.
[20] Robyn Klingler-Vidra and Steven Hai, ‘China’s chip industry is gaining momentum – it could alter the global economic and security landscape’ (The Conversation, 13 February 2024) <https://theconversation.com/chinas-chip-industry-is-gaining-momentum-it-could-alter-the-global-economic-and-security-landscape-222958> accessed 5 February 2025.
[21] Eleanor Olcott and Zijing Wu, ‘How small Chinese AI start-up DeepSeek shocked Silicon Valley’ (Financial Times, 24 January 2025) <https://www.ft.com/content/747a7b11-dcba-4aa5-8d25-403f56216d7e> accessed 5 February 2025.
[22] Jordan Schneider, ‘DeepSeek: What the Headlines Miss’ (ChinaTalk, 25 January 2025) <https://www.chinatalk.media/p/deepseek-what-the-headlines-miss> accessed 5 February 2025.
[23] George Hammond, Myles McCormick and David Keohane, ‘SoftBank and OpenAI back sweeping AI infrastructure project in US’ (Financial Times, 22 January 2025) <https://www.ft.com/content/48eb53a1-67ca-4509-8c62-401f0cf8b099> accessed 5 February 2025.
[24] Tamay Besiroglu, Sage Andrus Bergerson, Amelia Michael, Lennart Heim, Xueyun Luo and Neil Thompson, ‘The Compute Divide in Machine Learning: A Threat to Academic Contribution and Scrutiny?’ (arXiv, 2024) <https://arxiv.org/abs/2401.02452> accessed 5 February 2025.
[25] Nur Ahmed, Muntasir Wahed and Neil C. Thompson, ‘The growing influence of industry in AI research’ (2023) 379 (6635) Science <https://www.science.org/doi/full/10.1126/science.ade2420?casa_token=GSsE_kWLsR0AAAAA%3A09vfLkxisncrRkB-GzwMU3VNMuPYPgdHRLQM3BbEyy4IM5Fv95RCJoseWXheo-pjY8VjIAATFAK6> accessed 5 February 2025.
[26] Kenrick Cai, ‘AI Investors Are Wooing Startups With Massive Computing Clusters’ (Forbes, 14 February 2024) <https://www.forbes.com/sites/kenrickcai/2024/02/14/ai-investors-are-wooing-startups-with-massive-computing-clusters/> accessed 5 February 2025; and Aaron Mok, ‘Amid an A.I. Chip Shortage, the GPU Rental Market Is Booming’ (Observer, 23 October 2024) <https://observer.com/2024/10/ai-gpu-rental-startup/> accessed 5 February 2025.
[27] James Titcomb, ‘UK falls to record low in world supercomputer ranking as Starmer pulls funding’ (The Telegraph, 19 November 2024) <https://www.telegraph.co.uk/business/2024/11/19/uk-falls-to-record-low-in-global-supercomputer-ranking/> accessed 5 February 2025.
[28] For a discussion of this in the context of public sector LLM use, see Elliot Jones, ‘What is a foundation model?’ (Ada Lovelace Institute, 17 July 2023) <https://www.adalovelaceinstitute.org/resource/foundation-models-explainer/> accessed 5 February 2025.
[29] Pieter Haeck, ‘US limits on AI chips split EU’ (Politico, 14 January 2025) <https://www.politico.eu/article/eu-warns-back-against-us-artificial-intelligence-chip-export-china-limits/> accessed 5 February 2025.
[30] Ashwin Prasad and Satya S. Sahu, ‘Understanding the US’ New Framework for AI Diffusion’ (TechnoPolitik, 16 January 2025) <https://hightechir.substack.com/p/0x0e-understanding-us-new-framework> accessed 5 February 2025.
[31] ‘Britain’s Government Pulls the Plug on a Superfast Computer’ (The Economist, 22 August 2024) <https://www.economist.com/britain/2024/08/22/britains-government-pulls-the-plug-on-a-superfast-computer> accessed 31 October 2024.
[32] ‘China accelerates building of national computing power network’ (English.gov.cn, 2023) <https://english.www.gov.cn/news/202312/27/content_WS658b72afc6d0868f4e8e28ba.html>; Ning Zhang, Huabo Duan, Yuru Guan, Ruichang Mao, Guanghan Song, Jiakuan Yang and Yuli Shan, ‘The “Eastern Data and Western Computing” initiative in China contributes to its net-zero target’<https://www.sciencedirect.com/science/article/pii/S2095809924005058>; and ‘B-Roll, Video, Audio, Photos & Rush Transcript: Governor Hochul Launches First Phase of Empire AI, Powering Critical Research for the Public Good Just Six Months After FY25 Budget’ (New York State, 11 October 2024) <https://www.governor.ny.gov/news/b-roll-video-audio-photos-rush-transcript-governor-hochul-launches-first-phase-empire-ai> accessed 5 February 2025.
[33] Anthony K Cheetham and Ram Seshadri, ‘Artificial Intelligence Driving Materials Discovery? Perspective on the Article: Scaling Deep Learning for Materials Discovery’ (2024) 36(8) Chemistry of Materials <https://pubs.acs.org/doi/10.1021/acs.chemmater.4c00643> accessed 5 February 2025.
[34] Eleanor Olcott and Qianer Liu, ‘China offers AI computing ‘vouchers’ to its underpowered start-ups’ (Financial Times, 2024) <https://www.ft.com/content/9d67cda3-b157-47a0-98cb-e8e9842b2c90> accessed 5 February 2025.
[35] ‘People+AI’ <https://peopleplus.ai/occ> accessed 5 February 2025.
[36] Cloud credits are usually mechanisms to access cloud services, usually denoted in currency. For instance, a $5000 cloud credit provides access to cloud services from eligible vendors at the current market price, for up to $5000.
[37] Suraksha P and Himanshi Lohchab, ‘AI compute mission best served by marketplace model, say experts’ (Economic Times, 2024) <https://economictimes.indiatimes.com/tech/tech-bytes/marketplace-model-best-option-for-govt-to-offer-ai-compute-capacity-to-innovators-say-experts/articleshow/109169175.cms?from=mdr> accessed 5 February 2025.
[38] Ibid.
[39] For example, ‘Analysis: The UK Can’t Continue Its Shambolic Stop-Go Approach to Supercomputing’ (UCL, 9 August 2024) <https://www.ucl.ac.uk/news/2024/aug/analysis-uk-cant-continue-its-shambolic-stop-go-approach-supercomputing> accessed 31 October 2024.
[40] Central Statistics Office, ‘Key Findings Data Centres Metered Electricity Consumption 2023’<https://www.cso.ie/en/releasesandpublications/ep/p-dcmec/datacentresmeteredelectricityconsumption2023/keyfindings/> accessed 31 October 2024.
[41] EirGrid and SONI (System Operator for Northern Ireland), ‘Ireland Capacity Outlook 2022-2031’ (2022) <https://cms.eirgrid.ie/sites/default/files/publications/EirGrid_SONI_Ireland_Capacity_Outlook_2022-2031.pdf> accessed 31 October 2024.
[42] Environmental Protection Agency, ‘Ireland Projected to Fall Well Short of Climate Targets, Says EPA’ <https://www.epa.ie/news-releases/news-releases-2023/ireland-projected-to-fall-well-short-of-climate-targets-says-epa.php > accessed 31 October 2024.
[43] As found on pages 23-24 of ‘2024 Environmental Sustainability Report’ and page 6 of ‘Data Fact Sheet’’ (Microsoft, 2024) <https://www.microsoft.com/en-us/corporate-responsibility/sustainability/report> accessed 31 October 2024.
[44] ‘Freshwater Lochs’ (NatureScot) <https://www.nature.scot/landscapes-and-habitats/habitat-types/lochs-rivers-and-wetlands/freshwater-lochs> accessed 31 October 2024.
Image credit: luza studios