Safe beyond sale: post-deployment monitoring of AI
Building the information infrastructure to improve safe AI use
28 June 2024
Reading time: 14 minutes
The ‘irony of the information age’ is that the companies collecting the largest amounts of data are the ones least monitored by regulators.[i] Their AI technologies are based on oceans of data, but neither developers and deployers nor governments grasp AI’s waves impacting society.
AI is rapidly deployed, but information about deployment is missing.
Hundreds of millions of people use AI products in their daily lives. From mundane tasks, like drafting and summarising emails and writing code, to more complex or controversial applications like drafting court filings, creating songs that hit the charts or discovering new drugs (see also ERC 2023, Stanford HAI Index 2024), these systems can assume a broad range of functions. As of March 2024, 100% of Fortune 500 companies use AI systems like GPT-4, Claude 3, Llama 3 or Gemini.
This paints a picture of a new technology being rapidly deployed, against the backdrop of a general lack of awareness and understanding about how the technology is being used, and in what contexts.
The socio-economic implications of AI production, and especially of foundation models, are also alarming. A handful of companies – Google, Amazon, Microsoft, Meta, Apple, OpenAI and Anthropic – are building business models around utilising huge swathes of the data on the internet, negotiating exclusive access to large publishers’ proprietary data, and storing personal account data and user prompts to power their AI applications.[ii]
But do governments, for instance, know if entities that have a critical role in their country – like courts or utility companies – are using AI? Do governments track how AI changes societal systems, like the media or elections? Does the public know when they are subject to AI decisions? Are individuals aware of how AI algorithms influence their behaviour, for instance, their voting?
In most cases, the answer is no. But a malfunction in one of these critical settings will have severe repercussions for those directly affected and for society more broadly.
Being able to monitor if and how AI systems are used to carry out certain tasks is therefore a matter of urgency.
Indeed, even without critical malfunctions, AI is already affecting people’s lives, often without them knowing. AI-supported hackers access people’s online data; recruiters trust GPT-4 to screen applicants’ CVs and might reject them based on their names.
And pressure to use AI in the workplace has been rising, possibly leading to unsafe deployments. Tech-savvy colleagues can use large language models (LLMs) to get more work done faster, and there are some indications that employees not using AI are more likely to lose their jobs. Companies using AI save costs and sometimes outcompete others, but this is at a cost of lowering their safety standards.
This blog post reviews why post-deployment monitoring of AI models and applications is especially necessary. It maps out who, across the AI value chain, has access to the information that regulators and civil society need for monitoring mechanisms to be effective. And it looks at what a standardised system of control and reporting could look like. The final paragraphs make practical suggestions for implementing such a system.
Why does society need post-deployment monitoring and reporting?
Monitoring a product after its release on the market is common practice across industries in which public trust and safety are paramount. For example, the US Food and Drug Administration monitors population-level impacts of drugs. The EU’s Digital Services Act aims to monitor content moderation decisions and structural levels of misinformation.
The effectiveness of these measures varies across sectors: incident reporting in healthcare works best when coupled with standardised corrective actions. Accident monitoring and investigations by transport safety boards has sharply reduced fatalities across modes of transport, but only in high-income countries.
Compelling similar post-deployment monitoring and reporting for AI will therefore need to be developed over time to establish what works best. This may be especially important for AI, as many of the harms it might cause cannot be reliably anticipated before a model becomes available for use, either internally within companies or externally, when sold to the wider public on the market.
The performance of an AI system may change when used in new contexts, or when connected to other AI tools, like a web browser or calculator. Pre-deployment evaluations of capabilities, like asking an AI system high-school exam questions, cannot really assess the potential for society-level harms. There are many use cases that cannot be anticipated, like the consequences of an interaction of two AI systems. This means that ongoing testing and monitoring is necessary to understand impacts of specific deployments.
Clearly, the first step towards standardised post-deployment monitoring is to understand what type of information about an AI model and application should be monitored and reported.
What can be monitored and reported?
Figure 1: Information collection for governing AI[iii]
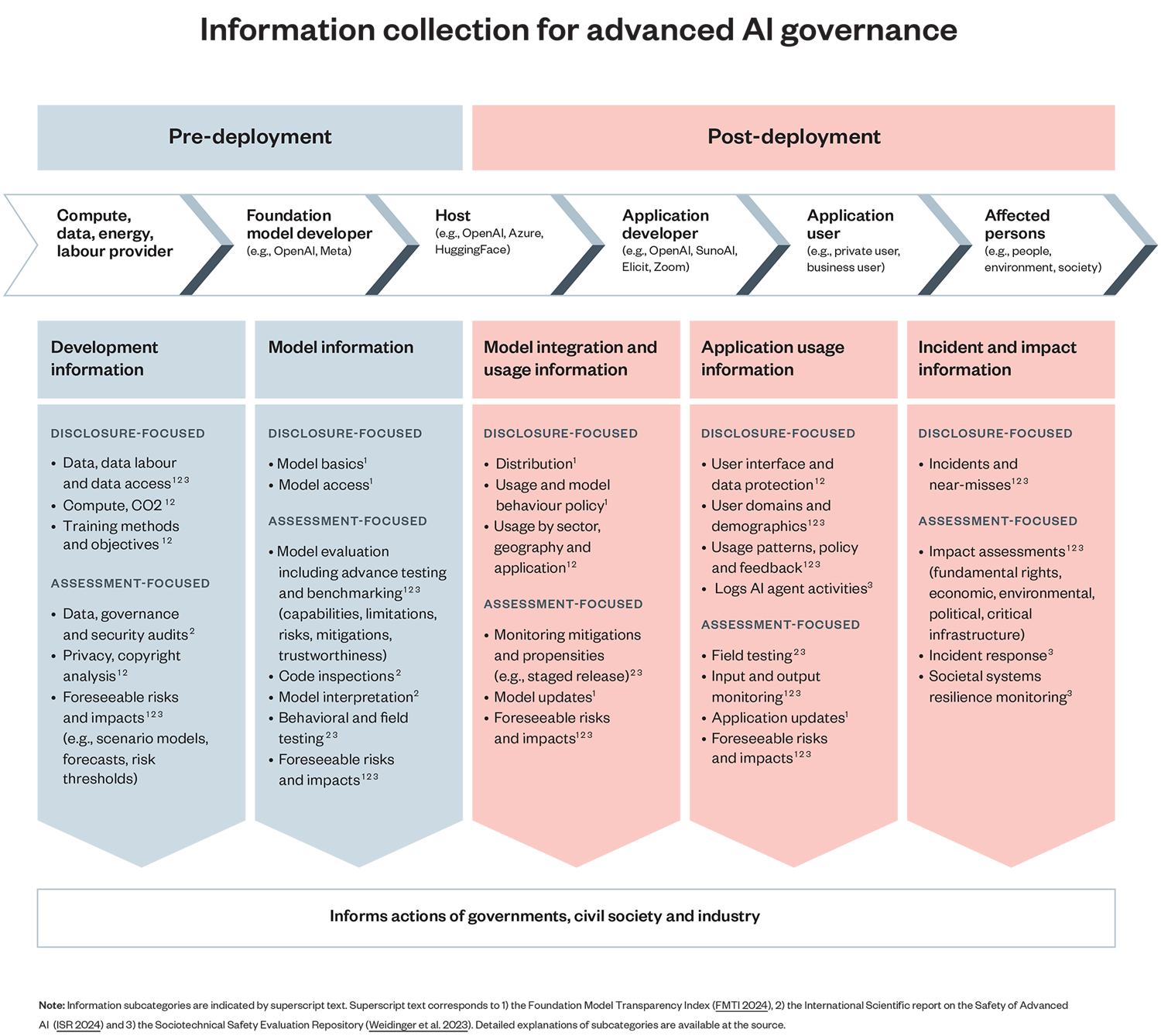
Along the AI value chain, there are different actors with relevant post-deployment information, as indicated in the figure:
- Hosts are cloud service providers or foundation model developers, who may make AI models available to downstream application providers or individuals.
- Application providers build and release applications that incorporate foundation models, like ChatGPT, Zoom AI Companion, Suno AI, Elicit or Duolingo.
- Application users are individuals, companies or public organisations using AI.
- Affected people are those whose lives are either directly impacted by a certain use of AI, for example when they are discriminated against at a job interview, or indirectly impacted, when an AI application results in changes in their environment and their access to material resources.[iv]
Now let’s unpack the three kinds of information that the diagram above identifies after a product has been deployed.
Model integration and usage information: Who uses AI?
Information on how a model is used and ‘integrated’ (that is plugged into an existing or new digital system) concerns how AI foundation models are made available on the market, and which application providers use them. This information can be organised according to the sectors in which providers operate, where they are based geographically and which applications they offer.
Ideally, model integration and usage information would be disclosed and shared with regulators. For example, regulators could monitor cross-cutting risks, like market concentration or fair access to AI products, by understanding which models are used in which sectors and geographical areas. This data could also inform decisions on how to regulate developers, hosts, application providers and deployers. For example, models integrated extensively in critical industries (such as infrastructure building) could be labelled as models with systemic risks under the EU AI Act.
Currently, such information (see also Similarweb 2024) is available only through high-level survey data and online activity. Business competition and privacy concerns limit hosts’ willingness to disclose information. However, in other sectors, sharing such information with governments is a regular practice. The UK’s Office for National Statistics continuously receives anonymised payment data from credit card providers and makes it publicly available for cross-industry economic monitoring and research. In the case of AI, government actors across the world are not yet empowered to access and decide about sharing such information.
Competition and markets authorities have been empowered over the last years to request integration information for the AI sector, but mostly in relation to specific inspections on company mergers .
Application usage information: How is AI used?
Application usage information includes information generated when a system is used. This includes, for example, monitoring the guardrails put in place (e.g. terms of use) and feedback (e.g. flagged misuse ) or socio-technical field tests. In addition, application usage data can be gathered by tracking AI watermarks and AI agent activity logs.
Ideally, developers, regulators and civil society organisations would be able to track instances of misuse of a model and severe malfunctions. In turn, this would help predict the possible impacts of different uses of the same AI model, and these predictions could inform which mitigation measures to adopt. For example, regulators might identify very consequential uses of a model like in hiring or critical infrastructure (see also UK ARIA 2024), and demand higher levels of reliability, safety and assured benefits.
There has not yet been a broad societal debate on usage monitoring for AI. Clearly, all organisations involved should address the concerns around privacy raised in relation to this type of data tracking. Especially in the context of increasingly authoritarian political dynamics, governmental scanning of consumers’ chat interactions with AI limits political freedom.
Currently, some AI application providers scan chat interactions to detect and terminate accounts of state-backed hackers. Taken to the extreme, this might resemble the strongly criticised proposal to scan WhatsApp messages through users’ devices, which was recently advanced by governments and providers.
Usage monitoring is already in place for financial algorithms (see also Chan et al. 2024, Aldasoro et al. 2024). An alternative way of accessing usage information may be voluntarily shared sample usage information, which is currently limited practice. Privacy-preserving ways of sharing usage data are currently tested to facilitate information access, while protecting user privacy.[v] There are also arguments in favour of restricting usage monitoring to businesses deploying autonomous AI agents or operating in high-risk industries.
Impact and incident information: Which harms are caused by AI?
Impact and incident information concerns adverse events and near-misses, broader societal effects, with a detailed understanding of why the incident occurred. This might include incident reporting, employment impacts, effects on socioeconomic inequality or on the resilience of societal systems. The latter might involve critical issues, such as the assessment of information pollution online that leads to widespread lack of trust – for example during electoral campaigns – which is hard to recover from.
Ideally, AI governance mechanisms improve outcomes for people and society. Unless incidents and impacts are monitored, it is impossible to understand whether AI governance mechanisms work, and if AI solutions are suited to a task. For this to be possible, private companies and public bodies will have to share detailed reports on incidents related to specific AI outputs, to understand their root causes and develop mitigation measures. Alongside this, researchers will have to assess societal resilience to understand the potential of a single incident to result in prolonged, cascading harms.
Currently, AI incidents are reported only via voluntary programmes, which are not as comprehensive as government-backed and mandated mechanisms that are in place in other industries. Similarly, AI’s impacts are poorly researched and predictions of future impacts are inconsistent and not sufficiently backed by evidence.
Figure 2: A mock up report with examples of the categories of information collected post-deployment
How to improve post-deployment monitoring and reporting?
There is a significant gap between the current state of post-deployment monitoring and reporting and the ideal scenario.[vi] We can attribute this to three key factors:
- Lack of overall post-deployment information and standardised processes to collect it. Given the uncertainty about AI’s risks, it is can be unclear what to measure and even developers, hosts and providers have only partial information[vii].
- Information asymmetry between AI companies, public bodies and civil society. AI companies have declined commitments to voluntary information sharing with public bodies and have only partly fulfilled transparency demands by civil society.[viii]
- Privacy and business sensitivity: governments need to establish when releasing information is truly in the public interest.
Post-deployment monitoring for AI is still a nascent field but both governments and large AI companies have responsibilities and a part to play in developing and improving the current state of the monitoring ecosystem. Here, we sketch their distinct roles.
What is the role of governments?
- Setting standards and compelling post-deployment monitoring and reporting. Current industry practice means there is a lack of transparency and shared evidence base.
- Ensuring access for a wider ecosystem of assessment to generate evidence. Broader public stakeholders (such as civil society organisations) are uniquely positioned to assess societal, economic and environmental implications of novel technologies like AI. Regulators should mandate AI companies to provide access for external assessors and vetted researchers.
- Funding post-deployment research.[ix]
- Building post-deployment monitoring capacity. As harms arise, sensitive information may be shared only with specific regulators, who will need to build internal capacity and mechanisms for compiling and sharing evidence.
What is the role of large AI companies and high-stakes application providers or deployers (such as governments themselves)?
- Following through on access and transparency commitments.[x] They should share information with regulators and publics, and grant access to models to regulators, civil society and users.
- Investing in post-deployment research. Companies must develop adequate assessments and monitoring measures internally, and support external researchers[xi] who have the resources and understanding to support post-deployment assessment.
- Building infrastructure for structured information sharing. Business claims around privacy, confidentiality and security will need to be considered. Privacy-preserving information sharing could help in some cases, but monitoring infrastructure of businesses by governments and civil society will remain a political question in many cases and will require a broad public debate.
- Clearly designating post-deployment responsibility within organisational structures. A senior member of staff with a dedicated support team should be made accountable for internal post-deployment risk procedures and sharing related information with relevant parties.
While post-deployment monitoring is not a silver bullet, current monitoring of AI does not deliver the benefits seen in other industries.
Current post-deployment monitoring does not yet reliably include AI usage and harm, or allow for evidence-based governance responses and corrective actions. Regulators, the AI safety institutes being set up in different countries (such as the UK’s AI Safety Institute) and large AI companies need to invest resources, develop standards, ensure access, share information and build technical infrastructure.
These efforts will build the information basis to help keep AI safe beyond sale.
We are grateful for input from Rishi Bommasani and Jamie Bernardi. This article is in part a summary of the PhD work of Merlin Stein at the University of Oxford.
Footnotes
[i] In the EU, the Digital Services Act and Digital Markets Act introduced partial regulatory monitoring in the last years.
[ii] Currently around 5-20%: The largest public training dataset DRBX contains 9 trillion words, estimates of words on the internet range from 100 to 200 trillion.
[iii] Each player along the value chain has access to different kinds of information. Each information category includes subcategories that are already available to respective value chain players (disclosure-focused) and categories that need dedicated resources (assessment-focused).
[iv] Directly affected persons or the environment; directly affected critical infrastructure, fundamental rights and property which then indirectly affects persons or the environment (see EU AI Act definition of serious incidents).
[v] Trusted flagger mechanisms empower local users with existing, distributed access to flag and correct AI outputs. In addition, PySoft allows data sharing via servers without researchers ever seeing the actual data.
[vi] These challenges suggest that monitoring of industry-focused AI and consumer-focused AI may need to be different. For the former, humans are not directly affected by AI. Thus, more information can be gathered and shared, to assess reliability and indirect human impacts. For the latter, privacy protection is essential. Thus, reduced monitoring and field testing of human interactions point towards the direct human impacts.
[vii] As defined in Figure 1, most companies have disclosure-focused information, but are lacking assessment-focused information.
[viii] Transparency on post-deployment downstream indicators is only partly measured and lower than pre-deployment upstream and model information.
[ix] Like the systemic safety grants of the UK AI Safety Institute.
[x] For example, as part of the White House Commitments or the Seoul AI Summit.
[xi] Small research grant programmes like OpenAI’s democratic inputs programme were rolled out, but more can be done by large AI companies who build the core products.
Related content
Safety first?
Reimagining the role of the UK AI Safety Institute in a wider UK governance framework
Safe before sale
Learnings from the FDA’s model of life sciences oversight for foundation models
Keeping an eye on AI
Approaches to government monitoring of the AI landscape