Networking with care part 1: Mapping ‘justice’
JUST AI aims to integrate and reflect on the many layers and different qualities of map-making, mapping and relationship construction
14 May 2021
In 2022, JUST AI moved to being hosted by the LSE. Learnings from this pilot project have contributed to greater understanding of the challenges and approaches to funding and hosting fellowship programmes, within the Ada Lovelace Institute and in the development of a broader data and AI research and funding community globally.
This is the first of a series of posts reflecting on different aspects of the mapping process. Here, we describe the conversation between quantitative literature mapping and qualitative critique, and the meaning of this conversation for the understanding of key data and AI ethics issues. This post describes our use of network methods to investigate the relationship between ‘data justice’ work and broader endeavours across the field of data and AI ethics.
We use network methods in our research as a way to describe different clusters of work occurring around data and AI ethics, and to identify the kinds of work capable of linking together different perspectives. We believe that in this urgent area of investigation, where issues have a broad impact, the separation and specialisation that often characterises scientific work might mean that the risks, harms and sociotechnical patterns of influence around data and AI are not discussed broadly between different kinds of practitioners and experts. This siloing influences how these ideas are talked about publicly as well. Given the significance of AI technologies across fields and in many areas of life, there is a lot at stake in terms of the quality and integration of discussions about data and AI ethics.
Understanding relationships of ideas
Quantitative and network-based literature mapping provides a way to assess the state of a research field by mapping and visualising it. Using social-network analysis techniques can include mapping the relationships between and prominence of institutions and individuals, as well as co-authorship (who writes with whom), to describe the relationships between people. Another set of techniques, including citation clusters, term co-occurrence mapping and topic modelling, looks at the thematic and conceptual structure of networks.
One of the maps of the field of data and AI ethics that we made based on one of these techniques – co-authorship relationships – looks like this:
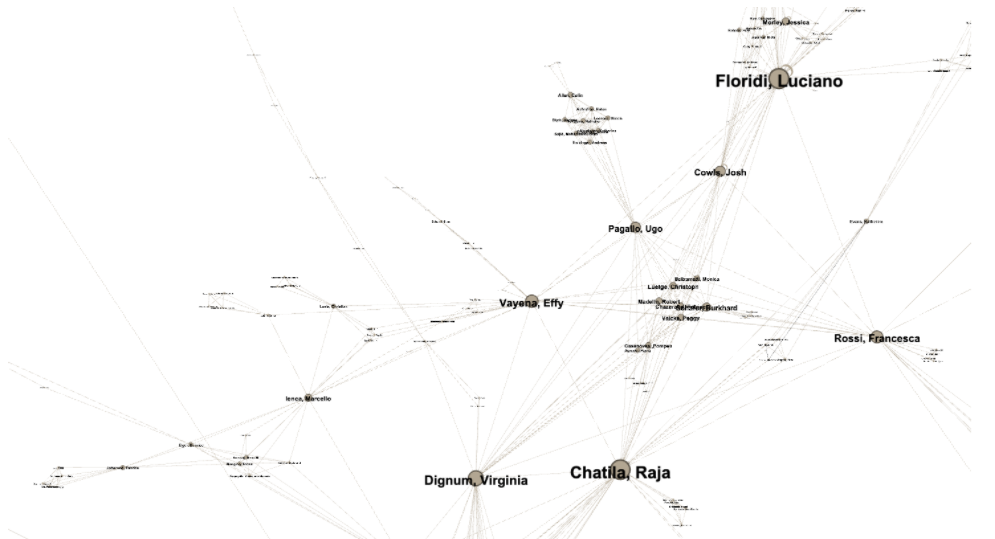
In this phase of literature mapping the JUST AI team drew on a deliberately restricted interpretation of data and AI ethics, which was operationalised into a search string that combined technical terms (‘data science’, ‘big data’, AI, ‘artificial intelligence’, ‘machine learning’, ‘robot*’, ‘autonomous system’, ‘automated decision’, ‘deep learning’, ‘autonomous vehicle’) with a small set of keywords to capture ethics (‘ethic*’ (the root word of ethic, ethics, ethical, etc.), moral and virtue) in the title, keywords or abstracts of papers found in major repositories: Clarivate’s Web of Science, the SpringerLink database, the digital library of the Association for Computing Machinery (ACM), as well as two preprint archives, the Social Science Research Network’s database and ArXiv. The search spanned the period between 2010 and March of 2021.
Collecting publication data like this might seem quite straightforward – after all it is digital ‘big data’ that you can collect through the search interfaces of repositories using automated methods. However, this data collection is a hugely labour-intensive exercise, with large amounts of manual effort going into the collection, aggregation, cleaning and harmonising of data drawn from different sources. In addition, some of these methods were new to our team, and we had to familiarise ourselves with the process. We also had to make important decisions about what to include in the final dataset, and we chose to make this decision after reviewing the abstracts of each paper individually to determine whether they were connected to AI/data ethics, or if the use of key terms was spurious, referring for example to obtaining ethics approval.

Following manual sorting, we used network methods to describe how people discuss different concepts and how specific (or critical) concepts create new conversations that link to larger discussions. For example, we were interested in exploring how an emerging concept that has become important to our project’s work, data justice, relates to conversations about AI ethics, as described above. To do this, we performed a similar search as before, but this time using only ‘data justice’ as an exact phrase, and mapped it against the papers in our larger AI ethics dataset that also address justice. Below we describe how this type of mapping helps to understand interdisciplinary conversations that are currently emerging.
Zooming in to ‘data justice’
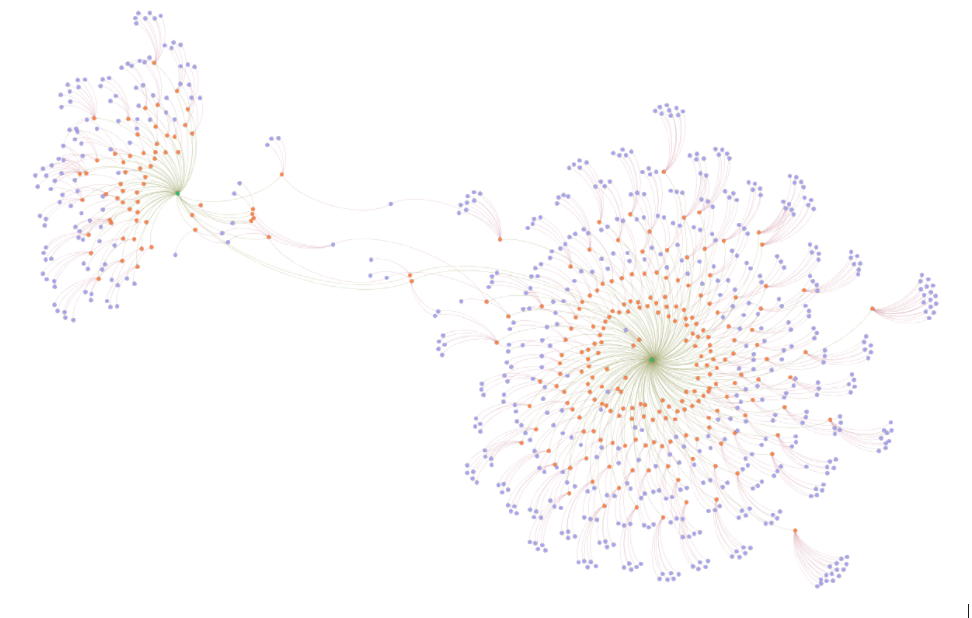
In the network visualisation we shared last week, we showed two clusters of papers centred around the concepts of ‘justice’ (as discussed within the literature on AI/data ethics) and ‘data justice’. These clusters are linked through a small number of scholars and papers, depicted in the network as an interconnected series of threads. These connections represent two possibilities. On the one hand, a small number of authors, such as Jo Bates or Emiliano Treré, make contributions to both discussions and therefore have different papers in both datasets. On the other hand, some papers, such as Linnet Taylor’s piece ‘What is Data Justice?‘ (2017), or Mamello Thinyane’s piece ‘Operationalizing Data Justice in Health Informatics‘ (2019), appears in both groups.
The connections of authors and papers to both groups suggest looking more closely at the interconnected threads (as described above) to find ways of envisioning the relationships between people and ideas. We read some of these papers to see what these links might be. For example, Emiliano Treré’s paper, ‘Exploring Data Justice’ (2019), describes the creation of the Data Justice Lab and Conference at Cardiff. This group of scholars and practitioners have been consciously working to redefine principles of data governance in line with principles of social justice. The Lab works collaboratively between scholars in law, policy, sociology and politics as well as with public-sector organisations. The lab holds a biannual interdisciplinary conference and publishes accessible white papers and reports along with academic articles. Data Justice Lab members have papers that appear across the two clusters – their approach is described as connective and interdisciplinary, and our description using network methods suggests that this indeed plays a significant role in defining a new approach and in sharing it broadly through strategic connections with other ideas. Given what is at stake in this research, interdisciplinary, connective research practices may well play a significant role.
Arguably, because our search for ‘ethics’ papers did not include the keyword ‘justice’ itself, some influential work is excluded from representation in the map above. This includes articles that our team recognise as significant to the field, even though they do not employ keywords in the same way. This points to the ways that these methods depend on how terms are defined at the start – you get out what you put in. We decided to separate ‘ethics’ and ‘justice’ in part because some scholars like D’Ignazio and Klein have noticed a bifurcation between ‘ethics’ and ‘justice’ and we were curious about what this might mean. It would equally be possible to define ‘justice’ as one of the key terms applying to ‘ethics’ – this could create a wholly different sort of network.
The multi-method conversations that JUST AI researchers have begun proceed from a spirit of generosity. This pays attention to the perspectives that different methodologies make possible, as well as to the different ways that researchers position their arguments. Together, these approaches highlight potential new conversations that can enhance work on data and AI ethics. We will be publishing our data openly on GitHub and inviting other researchers to work with it, as a way of generating other kinds of visualisations and other potential descriptions of the field.
Image credit: gremlin
Related content
Mapping AI and data ethics
Mapping the AI and data ethics field to understand the actors, issues and perspectives that constitute the space
JUST AI – Prototyping ethical futures for data and AI
Taking a creative, humanities-led approach to questions of AI ethics
To be seen we must be measured: data visualisation and inequality
How data, bodies and experience entwine.
What is visualised is realised: models and the fallacy of risk
How mathematical models of infection structure the messages people receive about risk and responsibility.